What is Predictive Analytics?
Predictive analytics is the practice of using historical data, statistical algorithms, and machine learning techniques to identify patterns and forecast future outcomes. Rather than simply describing what happened (descriptive analytics) or why it happened (diagnostic analytics), predictive analytics focuses on what is likely to happen next, and why.
It enables organizations to anticipate risks, uncover opportunities, and make proactive decisions based on data-driven insights.
Why Predictive Analytics Matters
In a world driven by data, reacting is no longer enough—enterprises need to stay ahead of the curve. Predictive analytics empowers organizations to do just that by turning raw data into forward-looking intelligence. Whether it’s forecasting customer behavior, anticipating equipment failure, or identifying emerging fraud risks, predictive models help teams act before the moment demands it.
According to Gartner, by 2026, over 60% of enterprises will have embedded predictive capabilities into their decision-making processes, fundamentally changing how strategy, operations, and risk are managed.
Key Components of Predictive Analytics
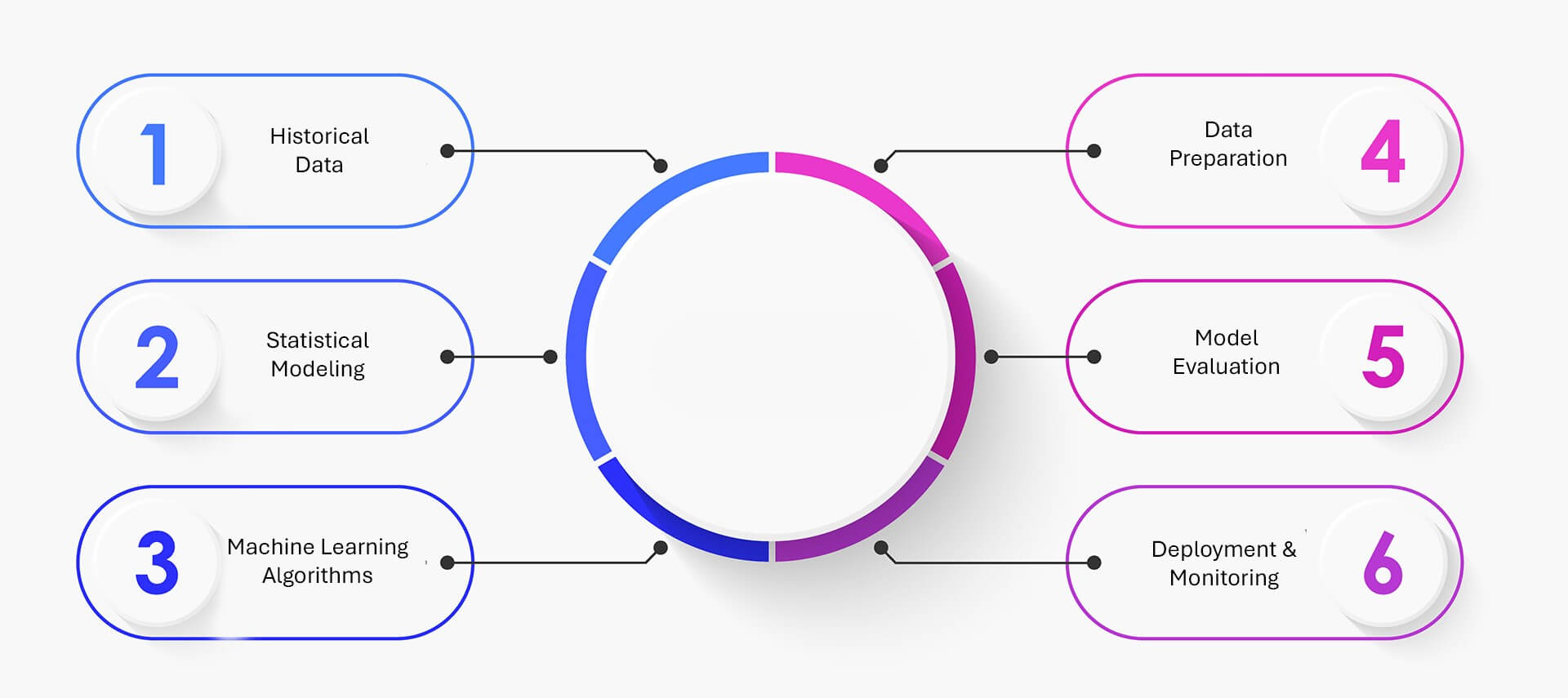
- Historical Data: Clean, structured datasets that serve as the foundation for analysis.
- Statistical Modeling: Techniques such as regression, time series analysis, and decision trees used to detect trends and correlations.
- Machine Learning Algorithms: Adaptive models that improve over time by learning from new data (e.g., random forest, gradient boosting, neural networks).
- Data Preparation: Data cleansing, normalization, and feature engineering to ensure high-quality inputs.
- Model Evaluation: Metrics like accuracy, precision, recall, and AUC are used to assess and validate the model’s performance.
- Deployment & Monitoring: Integrating predictive models into business applications and monitoring them to avoid drift or decay over time.
Challenges in Predictive Analytics
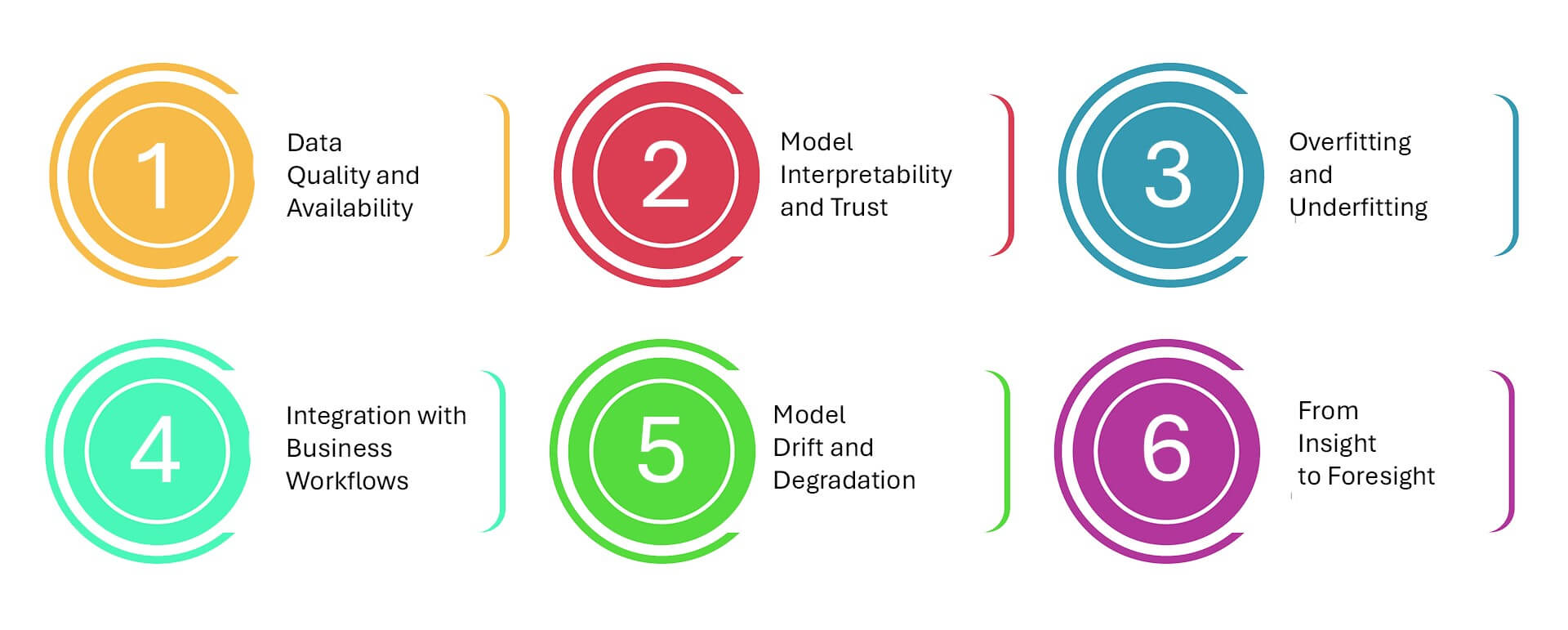
Data Quality and Availability
Predictive models are only as good as the data that feeds them. Incomplete, inconsistent, or biased data leads to unreliable forecasts.
What to do: Invest in robust data governance practices, automated data cleansing, and metadata management. Ensure data is complete, timely, and contextually rich before feeding it into models.
Model Interpretability and Trust
Complex machine learning models can operate like black boxes, making it difficult for stakeholders to trust or understand predictions.
What to do: Use explainable AI (XAI) frameworks to highlight how decisions are made. Provide clear model documentation, visual outputs, and business context for transparency.
Overfitting and Underfitting
Poorly tuned models may either learn noise instead of signals (overfitting) or fail to learn key patterns altogether (underfitting).
What to do: Split data into training, validation, and test sets. Use techniques like cross-validation and regularization to balance generalization and accuracy.
Integration with Business Workflows
Predictive models often sit outside of operational systems, limiting their real-world impact.
What to do: Deploy models via APIs or embed them directly into enterprise platforms like CRM, ERP, or BI dashboards. Automate decision triggers using the model’s output.
Model Drift and Degradation
Business environments evolve. Without ongoing calibration, predictive models lose relevance as data patterns shift.
What to do: Set up continuous monitoring, feedback loops, and model retraining schedules. Use drift detection techniques to track when re-training is necessary.
From Insight to Foresight: The Expanding Impact of Predictive Analytics
Predictive analytics has moved beyond being a mere data science function—it is now the intelligence layer fueling next-generation enterprise strategy. As a core component of AI architecture, it drives real-time risk mitigation, personalized automation, and operational forecasting across sectors like finance, healthcare, retail, and manufacturing.
Predictive analytics turns historical data into a decision-making advantage by enabling systems to recognize patterns, anticipate outcomes, and recommend actions. In AI pipelines, it sharpens accuracy and responsiveness, helping algorithms learn and adapt meaningfully to new contexts.
More importantly, predictive analytics is becoming a strategic differentiator in the shift toward proactive, data-driven operations. When embedded into governance frameworks and aligned with compliance, it ensures that intelligence doesn’t come at the cost of control. It empowers leaders to replace reactive decisions with forward-looking confidence, balancing agility with accountability in every corner of the enterprise.
Predictive analytics is not just about prediction—it’s about preparation. It transforms raw data into action, empowering enterprises to operate proactively in uncertain environments. With the right data, tools, and oversight, predictive analytics becomes a competitive differentiator—shaping strategy, minimizing risk, and unlocking new growth opportunities.
Because in today’s digital economy, knowing what might happen next isn’t a luxury—it’s a necessity.
Getting Started with Data Dynamics:
- Learn about Unstructured Data Management
- Schedule a demo with our team
- Read the latest blog: UK’s Data Protection Reforms: Navigating Compliance Risks and Redefining Data Management for a New Era