What is Data Sprawl?
Data sprawl refers to the uncontrolled growth and distribution of data across an organization’s digital environment. This phenomenon occurs when data is created, stored, copied, and moved across various locations—clouds, on-premises servers, endpoints, collaboration platforms, SaaS applications, and shadow IT—without centralized oversight or governance.
In simpler terms, data sprawl is the digital equivalent of clutter. And in the enterprise context, this clutter can have serious consequences, ranging from security vulnerabilities and compliance risks to storage inefficiencies and AI model failures.
Why Does Data Sprawl Matter?
The shift toward hybrid work, rapid cloud adoption, and the explosion of unstructured data have created a perfect storm for data sprawl. IDC predicts that by 2025, more than 80% of enterprise data will be unstructured, scattered across silos, and largely invisible to IT teams. According to IBM X-Force, 68% of cyberattacks now target unstructured data, precisely because it’s often overlooked, unprotected, and improperly governed.
Data sprawl is no longer just a storage or compliance concern—it’s a barrier to digital transformation, AI readiness, and operational resilience.
Key Characteristics of Data Sprawl
- Uncontrolled Data Growth: Data is being generated at unprecedented rates across devices, departments, and applications, without defined retention or lifecycle policies.
- Siloed Storage: Data resides in fragmented silos—file shares, cloud buckets, email servers, collaboration tools—often inaccessible to centralized governance systems.
- Shadow IT and Unsanctioned Applications: Employees use unauthorized tools to share and store data, leading to duplication, loss of context, and security gaps.
- Redundant, Obsolete, and Trivial (ROT) Data: A significant portion of sprawl consists of outdated or low-value data, which consumes storage and poses unnecessary risks.
- Limited Visibility and Traceability: Organizations lack a unified view of where sensitive data resides, who owns it, and how it is being used or shared.
Technical Implications of Data Sprawl
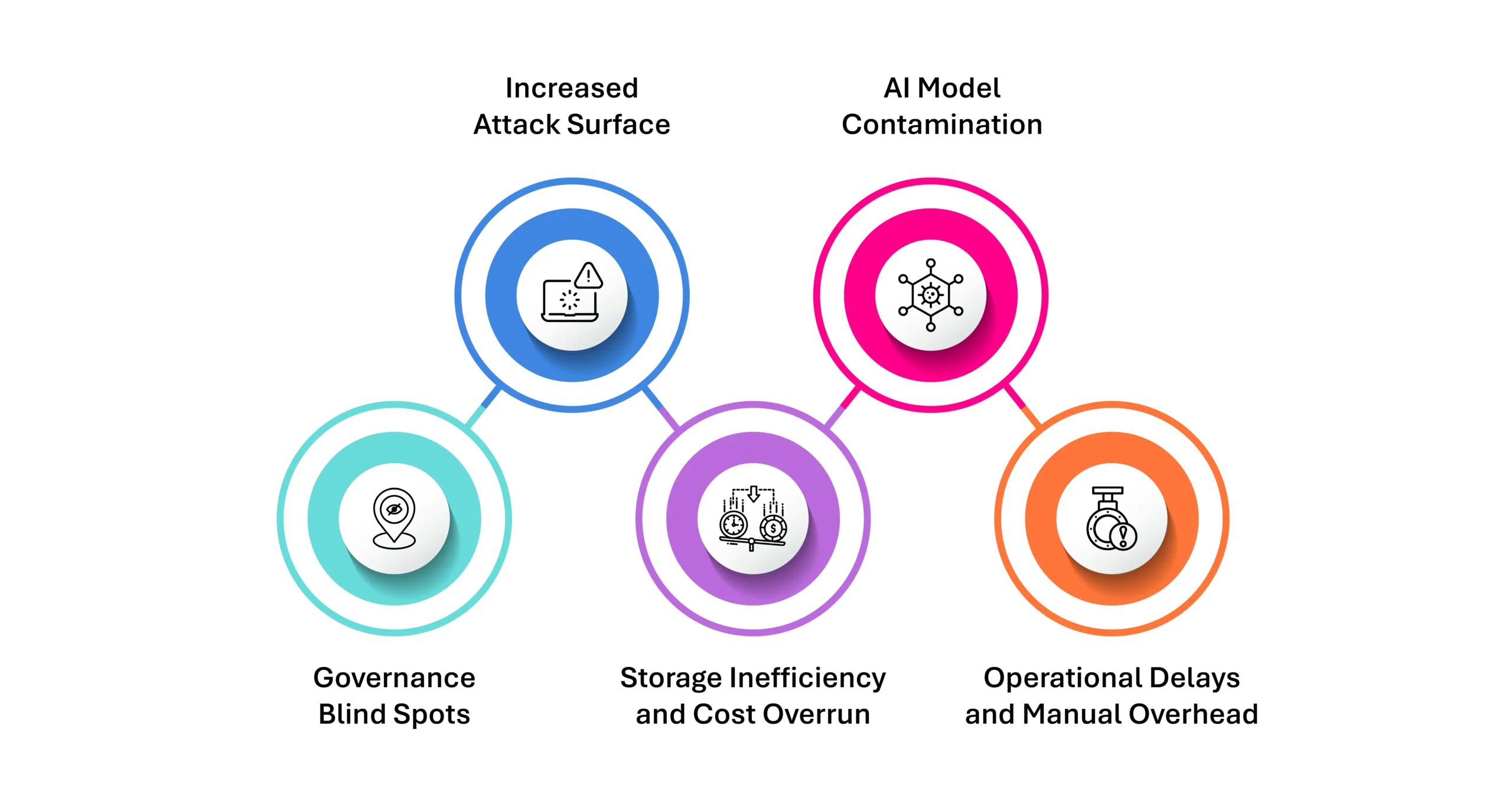
1. Governance Blind Spots
Without centralized metadata mapping and classification, data sprawl leads to unknown unknowns—data assets that exist but are invisible to governance teams. This creates gaps in compliance with regulations such as GDPR, HIPAA, and India’s DPDP Act.
2. Increased Attack Surface
Scattered data increases the number of potential entry points for threat actors. Orphaned files, stale backups, and unsecured cloud buckets become easy targets for ransomware, insider threats, or accidental exposure.
3. Storage Inefficiency and Cost Overrun
Sprawled data leads to uncontrolled storage growth and redundant storage tiers. Enterprises may unknowingly store duplicate datasets across environments, leading to inflated infrastructure and cloud costs.
4. AI Model Contamination
When training AI models, feeding in incomplete, redundant, or irrelevant data from sprawled sources can degrade model accuracy. It also raises the risk of biased outputs, hallucinations, or ethical violations due to unvetted training datasets.
5. Operational Delays and Manual Overhead
Data sprawl slows down IT teams who must spend excessive time locating, cleaning, or moving data. It also delays analytics pipelines and introduces friction into business decision-making processes.
How to Contain Data Sprawl: A Modern Approach
Containing data sprawl requires a unified strategy across visibility, control, and action. Here’s how modern enterprises are tackling it:
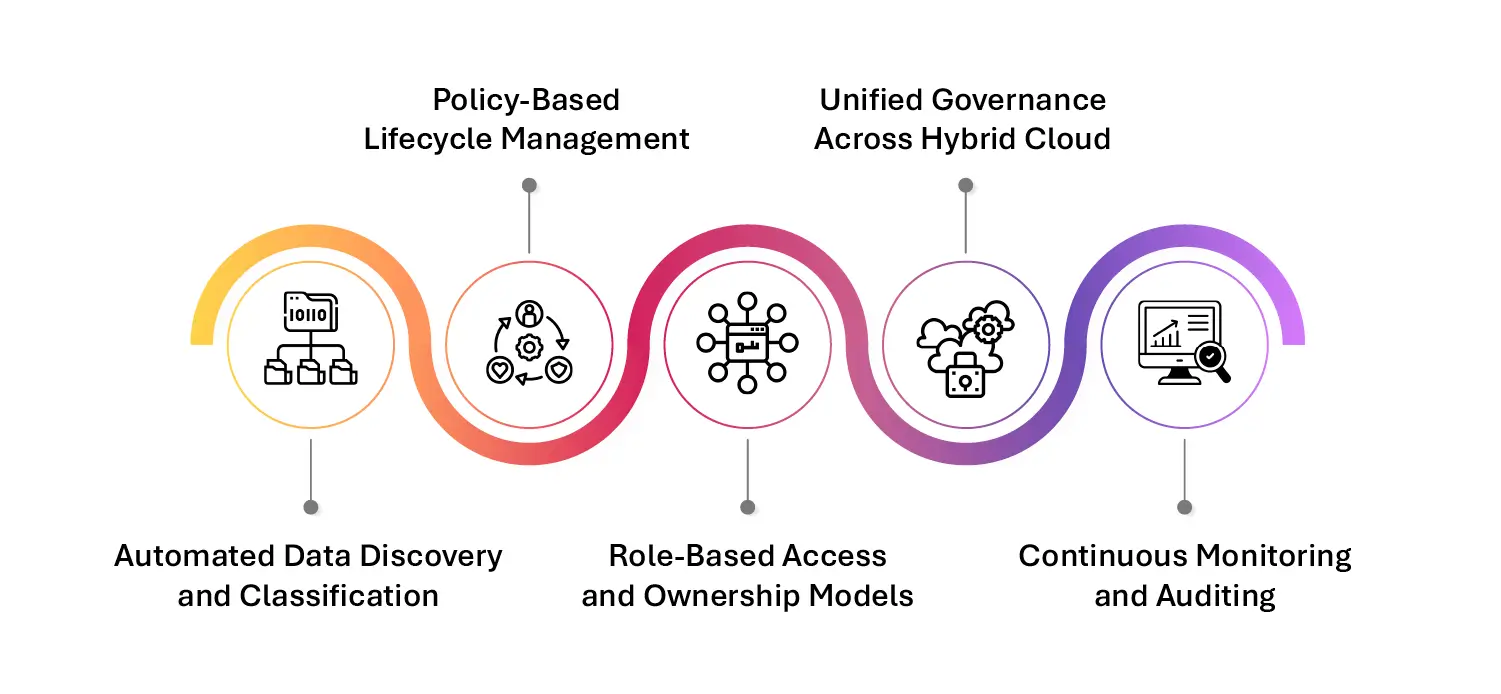
- Automated Data Discovery and Classification: Use AI and metadata analytics to scan enterprise environments and identify sensitive, redundant, or critical data.
- Policy-Based Lifecycle Management: Define rules for data retention, archiving, movement, and deletion to prevent unnecessary accumulation.
- Role-Based Access and Ownership Models: Empower data owners to manage their own data domains, reducing the burden on central IT while enhancing accountability.
- Unified Governance Across Hybrid Cloud: Implement tools that operate across on-prem, multi-cloud, and SaaS platforms to ensure consistency in policy enforcement.
- Continuous Monitoring and Auditing: Integrate data lineage tracking, anomaly detection, and audit logging to proactively manage sprawl as environments evolve.
As generative AI, edge computing, and real-time analytics continue to scale, the data sprawl problem will only intensify. The future lies in self-service data management ecosystems that give control back to data owners while embedding automation, governance, and security into the fabric of data workflows.
In this future, managing sprawl won’t be an afterthought—it’ll be a foundational pillar for operational agility, data sovereignty, and ethical AI development.
Getting Started with Data Dynamics:
- Learn about Unstructured Data Management
- Schedule a demo with our team
- Read the latest blog: AI Is Only as Secure as the Data You Feed It. Is Yours Truly Ready?