Importance of Data Quality Management throughout Cloud Migration Lifecycle for Energy Industry.
The energy sector is rapidly evolving. As per one of the recent reports by Technavio, the oil and gas sector alone will see growth by USD 8.37 billion during 2021-2025, registering a CAGR of 19.40%. In today’s world of digitization, growth is synonymous with the generation of data. Believe me, when I say this, today’s energy industry is generating heaps of field data!
In order to manage their data efficiently and gain maximum insights from it, they need a solution that will help them break free from the cumbersome conventional data management mechanisms and migrate them to less expensive and agile infrastructure. The answer is simple – Cloud Computing.
According to Gartner’s 2021 CIO Survey, 97% of oil and gas companies have defined their digital investment programs and the business value they will generate by 2025. This clearly implies the need to migrate business operations, supporting data, and some core functions on the Cloud.
The Energy industry relies on an array of massive databases comprising of data generated from various data points such as transmitters, detectors, sensors, logging systems, and more. The industry’s objective clearly is to utilize this data to render business benefits. Moving this field data on the Cloud can empower enterprises to optimize their capabilities by extracting actionable & real-time insights, enabling predictive analytics, and ensuring maximum safety.
The path towards an intelligent, error-free, and secure cloud migration journey
Moving to the Cloud is a big decision for any company. Typically, this decision is purely financial. Ease of deployment or faster deployment can affect a company’s ability to react to the market with either expansion or contraction. In some cases, having a predictable monthly cost can help the revenue goals and budgeting.
Data quality matters because it can affect everything from cost to speed and risk. I have helped several companies either create their own managed Cloud or go to a fully hosted cloud solution. Their methodology and reasons varied greatly.
- One energy company was evacuating all their worldwide data centers.
- An organization was upgrading old technology, and instead of purchasing new, they moved it to the Cloud.
- Significant manufacturer splitting in business was moving to a variety of solutions.
The decision-making on what data to move, how to move it, and why it should be moved also varied. Much of this depended on where the company was in its data management journey. It’s also based on the type of data being moved.
The following discussion illustrates the crucial points towards successful cloud migration.
A simple, easy-to-follow data lifecycle management policy is the first step
Devising a simple data lifecycle management policy relies on two factors:
- Business unit needs
- Corporate goals
An example of this is archiving data or deleting completely. Another might be deleting data that hasn’t changed or touched, say seven years or more.
The general stat for Structured data vs. Unstructured data size is 25% vs. 75%. Because structured data is highly managed, it tends to have a smaller footprint and a higher quality level. On the other hand, unstructured data is created by individual users or applications.
In the case of applications, there is usually a data policy on what files to keep and how long. In the case of individual users, much of the data is generated either become part of a business process or forgotten. Even if the company is not moving to the Cloud, managing this data efficiently can save money and reduce risk.
Before we move to the Cloud, developing a tiered approach for files and their data is essential. Establishing criteria for what goes to what tier, what might need to be deleted, or needs additional protection because of its criticality.
One critical step is to analyze metadata. Metadata is an external set of information about the file and its contents in files, for instance, creation date, modified time, file owner, file extension, file ACLS, and 100 other properties. The internal data or file contents can be critical to understating. Having the ability to gather metadata and understand the risk in the file contents increases the overall quality of data.
Some examples of metadata improvement searches:
- Find all the files from a retired application. E.G *.nsf = old Lotus notes files
- Find any file with an identified ACL. Ex. user_deny ACL created for security but flawed
- Find any file with a combination of
- Last modified older than seven years
- Last access time older than seven years
- File type = bck
The all-encompassing four-step approach critical towards efficient data management is as below:
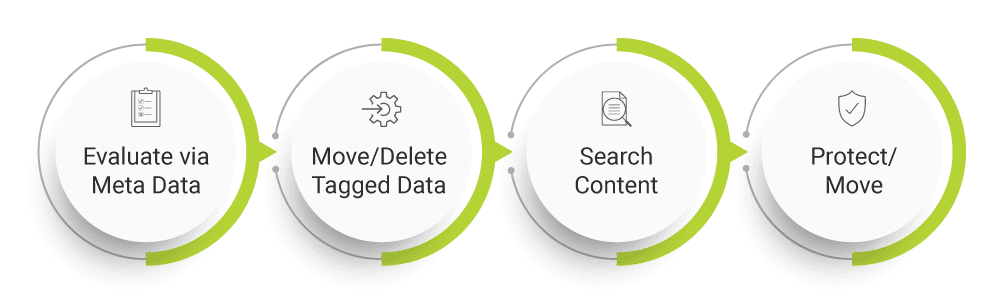
Figure 1 – Data Quality Process
Once the data management group has completed the above steps and establishes guidelines, it becomes easy to pare down the data for what matters to the business. There is a choice to move data based on the metadata or further secure the data with targeted deep risk analysis.
What is targeted, deep risk analysis
Targeted, deep risk analysis performs a streaming operation on each file. It then uses pattern matching assisted by AI to find all manner of Personal Identifiable Information that can put a company at risk if exposed. The remediation of the data can then be dealt with in one of several ways:
- Left alone
- Apply targeted security ACL’s
- Move the files to another tier of file storage
- Move the files to an S3/Object/Blob based system
What data needs to be found?
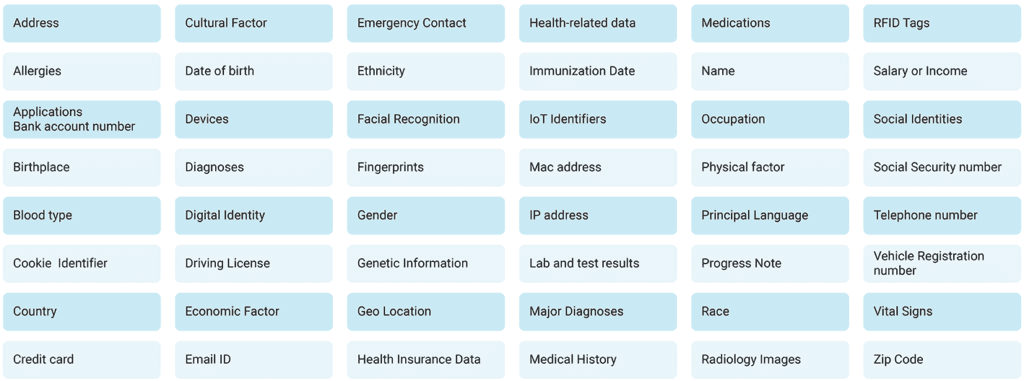
Figure 2- Table of PII data
The output of targeted, deep risk analysis comes in the form of lists of files that contain the information that will need to be acted on. Properly executed, the process of finding the data with risk has one or more approvers to place or protect it. Each movement must be tracked to ensure compliance with the data governance policies.
The critical part of the process is approvals because data ownership is typically defined at a business unit level. Most times, the people who approve the data management are not making the discovery.
The one-stop technology solution
Data Quality Management and Data Governance are not just about defining what high-quality data looks like but also about devising ways for tracking and working with that data. It is a continuous process and goes beyond just data migration. Culling data is not enough. Finding data that puts your organization at risk is a necessary part of the journey to the Cloud and is essential when you are already on the Cloud.
Data Dynamics’ Unified Unstructured Data Management Platform plays a crucial role in improving conventional, unconventional, and midstream operations in the industry. This systematic data quality management platform ensures the issues are addressed as they arise and provides security and compliance of the migrated data. It continues these efforts post-migration as well to address issues and remediate them immediately. The platform can be integrated into any business process targeted at data governance and data quality initiatives. With Data Dynamics, enterprises in the Energy sector can efficiently manage, process, and secure immense datasets from upstream, midstream, and downstream operations.
If you’d like to know more about how Data Dynamics can help elevate your Data Quality journey, reach out to us – solutions@datdyn.com. You can also visit our website at www.datadynamicsinc.com or click here to book a meeting.