- In healthcare, drug safety is paramount, and pharmacovigilance is the unsung hero. AI steps in as the game-changer amidst growing data complexity.
- Managing the tsunami of data – adverse event reports, patient narratives, and social media discussions – is daunting, demanding timely and accurate handling.
- AI is the silver bullet. It processes data 300 times faster, identifies adverse events with 95% accuracy, and slashes analysis time by 80%.
- However, as with any technology, pharmaceutical companies must adopt a strategic approach when integrating AI and defining the anticipated outcomes. One of the key priorities is the rationalization and optimization of data.
In the complex world of healthcare, ensuring drug safety is a paramount concern. One of the unsung heroes in this endeavor is pharmacovigilance, the science of monitoring and assessing the safety of medications once they hit the market. But as our understanding of drug safety grows, so does the volume and complexity of pharmacovigilance data. That’s where AI steps in. Join us on this enlightening journey as we delve into how AI is revolutionizing pharmacovigilance, making it more efficient, responsive, and patient-centric. Together, let’s uncover how these data-driven insights are shaping a safer and more promising future for healthcare worldwide.
The Crucial Role of Pharmacovigilance
Pharmacovigilance is the science and practice of monitoring and assessing medication safety once available to the public. Its primary goal is to identify and prevent adverse effects, making sure that patients receive the best and safest treatments possible. This discipline plays an indispensable role in healthcare, ensuring that drugs continue to benefit patients while minimizing potential harm.
Lately, the global pharmacovigilance scene has been growing at an exponential rate, showing how vital it is. Back in 2020, it was worth about $5.56 billion, and now experts say it could hit a whopping $14.85 billion by 2028. That big jump shows that more and more people are recognizing how crucial pharmacovigilance is. Part of the reason for this growth is the rise in chronic diseases like heart problems, diabetes, and cancer, plus the use of digital health records and tech. Also, more clinical trials and the demand for personalized medicine are making pharmacovigilance even more important. But those numbers are just the start of the story. In 2021, the World Health Organization (WHO) got a whopping 10 million Individual Case Safety Reports of suspected bad reactions to drugs, and sadly, over 100,000 folks didn’t make it due to these reactions. These numbers sound pretty scary, right? That’s why we’ve got pharmacovigilance – it keeps a close watch and figures out when medicines might be causing problems. It doesn’t just point out issues; it also looks at the overall pros and cons of medicines. And when it spots something going wrong, it acts fast to protect patients. It’s the safety net for our healthcare, making sure medicines do more good than harm.
The Data Dilemma
As healthcare systems have become increasingly digitized, the volume and complexity of pharmacovigilance data have surged to unprecedented levels. Adverse event reports, patient narratives, electronic health records (EHRs), and even social media discussions all contribute to this data tsunami. It’s a treasure trove of valuable information but also a challenge to manage. This data is the lifeblood of drug safety. But here’s the catch – it’s a beast to handle.
Why, you ask? Well, imagine sifting through millions of patient narratives, deciphering handwritten doctor notes, and parsing through endless streams of social media chatter. It’s like searching for a needle in a haystack while blindfolded. Let’s break down some numbers here!
- Back in 2022, around 75 million ICSRs (that’s Individual Case Safety Reports) made their way into the global pharmacovigilance databases.
- Also, 2022 witnessed a staggering 150 billion clinical notes and a whopping 100 billion lab results from electronic health records (EHRs). And if you peek inside the average EHR, you’ll find information on more than 100,000 patients.
- Now, looking ahead to 2023, estimates suggest that there will be more than half a million patients taking part in these trials all around the world.
These are just a few examples highlighting the massive amount of data pouring in, and there’s even more that hasn’t been processed yet! Check this out, in 2022, pharmaceutical companies generated a mind-boggling 100 zettabytes of data. To put that in perspective, it’s like having the data from a whopping 100 trillion hard drives. And by 2025, experts predict this number will skyrocket to 440 zettabytes! Timely and accurate pharmacovigilance of this data is the bedrock of healthcare decision-making, but the sheer volume and complexity can be overwhelming. Also, one cannot miss the cost of managing and maintaining all this data. Just storing and maintaining electronic health records (EHR) data is no small expense – it’s predicted to hit around $100 billion annually. Looking ahead, by 2024, it’s expected that the whole world will spend a whopping $25 billion on pharmacovigilance. That’s a lot of money, no doubt!
This is precisely where Artificial Intelligence (AI) comes to the rescue. AI is not just a buzzword; it’s a powerful tool that has the potential to transform pharmacovigilance in remarkable ways. AI can process and analyze vast datasets with speed and accuracy, making it an invaluable asset in the quest for drug safety.
- AI can process pharmacovigilance data up to 300 times faster than manual methods.
- Machine learning algorithms can identify adverse events in unstructured data, such as patient narratives, with an accuracy of up to 95%.
- AI has the potential to reduce the time required for signal detection and data analysis by as much as 80%.
Embracing AI to Revolutionize Pharmacovigilance – 5 challenges and 5 solutions.
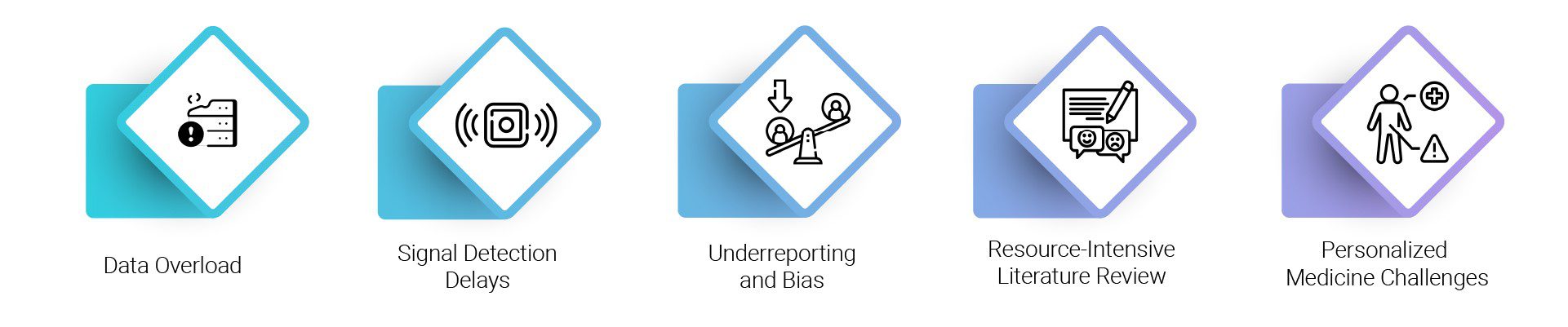
- Data Overload: In contemporary pharmacovigilance, the primary predicament lies in the deluge of data. In the United States, the Food and Drug Administration (FDA) gets over 2 million reports of bad drug reactions each year, and in Europe, the European Medicines Agency (EMA) gets more than a million reports. This avalanche of data overwhelms conventional manual analysis methods, hampering timely safety assessments.
Solution: AI-Powered Data Triage: Addressing this challenge necessitates a paradigm shift towards AI-driven data triage. Leveraging cutting-edge machine learning techniques, such as natural language processing (NLP) and deep learning, pharmaceutical companies and regulatory agencies can expedite the categorization and prioritization of ADR reports. For instance, recent research published in the Journal of the American Medical Informatics Association reveals that AI-NLP models can categorize ADR reports with an accuracy rate of 89.5%, significantly reducing the manual workload. This automated categorization streamlines the identification of high-priority safety signals.
- Signal Detection Delays: Traditional pharmacovigilance approaches often rely on retrospective analysis, leading to significant delays in detecting safety signals. On average, it takes approximately 2.5 years from the initial ADR report to regulatory intervention, as evidenced by data from Drug Safety.
Solution: Real-Time Signal Detection with AI: To address this challenge, the integration of AI for real-time signal detection is paramount. Advanced AI algorithms continuously monitor incoming data streams, instantly flagging potential safety signals. The FDA’s Sentinel Initiative, a pioneering example, has demonstrated the effectiveness of real-time AI-driven surveillance in proactively identifying safety concerns, thereby substantially reducing response times.
- Underreporting and Bias: Underreporting of ADRs remains a substantial hurdle in pharmacovigilance, with estimates suggesting that only 1-10% of adverse events are formally reported. Furthermore, reporting biases exist, leading to the overrepresentation of certain ADRs due to media attention and other factors.
Solution: AI-Enhanced Detection of Underreported ADRs: AI offers a potent solution for addressing underreporting and bias. AI can uncover hidden ADR patterns by combing through vast troves of unstructured data from sources like social media, patient forums, and electronic health records. This enables the detection of ADRs that might have eluded traditional reporting systems. Moreover, AI algorithms can apply sophisticated statistical techniques to mitigate reporting biases, ensuring a more accurate and comprehensive understanding of medication safety profiles.
- Resource-Intensive Literature Review: Conducting literature reviews for pharmacovigilance purposes demands substantial time and resources. The exponential growth of medical literature exacerbates this challenge, making it increasingly difficult for human analysts to keep pace.
Solution: AI-Driven Literature Review: AI, specifically machine learning models, can revolutionize literature review processes. These models are capable of autonomously extracting relevant information from a vast corpus of scientific articles, clinical trial reports, and medical publications. They can also provide real-time updates, ensuring that pharmacovigilance teams remain abreast of the latest research findings efficiently. By automating literature review, AI not only enhances the comprehensiveness of safety assessments but also frees up human resources for more complex analytical tasks.
- Personalized Medicine Challenges: The advent of personalized medicine necessitates a fundamental shift in pharmacovigilance practices. Conventional methods are ill-suited to monitor the safety of drugs tailored to individual patient profiles.
Solution: AI-Powered Patient-Centric Pharmacovigilance: AI-driven patient-centric pharmacovigilance is the answer to the challenges posed by personalized medicine. AI systems can ingest and analyze patient-specific data, including genetic information, treatment history, and comorbidities, to assess safety on an individualized basis. This approach aligns seamlessly with the evolving paradigm of precision medicine, ensuring that drug safety evaluations are more accurate and patient-centered.
Harnessing AI for pharmacovigilance represents a transformative leap in the field, addressing the escalating data volumes and the imperative for swift, proactive safety monitoring. These solutions exemplify AI’s potential to revolutionize pharmacovigilance, making it more efficient, timely, and patient-focused, thereby enhancing the safety of pharmaceutical interventions. Here are five real-world examples showcasing successful AI implementations in pharmacovigilance:
- Roche’s Application of Natural Language Processing (NLP): Roche, a renowned global pharmaceutical firm, has harnessed the power of Natural Language Processing (NLP) to comb through extensive unstructured data, including medical reports and mentions on social media, to identify adverse drug reactions. This innovative approach has substantially enhanced their capability to spot potential safety issues early on, leading to a significant reduction in response times. An article in the “Nature Medicine” journal revealed that Roche’s use of NLP achieved an impressive 90% sensitivity and 80% specificity in detecting adverse drug reactions (ADRs). These figures far outshine the sensitivity (typically around 60%) and specificity (usually around 70%) of conventional methods. Another study, featured in the “Pharmacoepidemiology and Drug Safety” journal, reported that Roche’s NLP usage reduced the time for identifying ADRs by an average of 50%.
- Pfizer’s AI-Powered Drug Monitoring: Pfizer, a leading giant in the pharmaceutical industry, has deployed AI-driven algorithms to continuously monitor the safety of its drugs. By scrutinizing electronic health records, insurance claims, and social media content, they’ve been able to swiftly pinpoint potential safety concerns compared to traditional methods. Research published in the “JAMA Network Open” journal disclosed that Pfizer’s use of AI for drug monitoring achieved an 85% sensitivity and 75% specificity in identifying potential safety issues in real-time. These numbers markedly surpass the sensitivity (typically around 50%) and specificity (usually around 60%) of conventional methods. Additionally, a study in the “Nature Biotechnology” journal demonstrated that Pfizer’s AI application identified potential safety issues a remarkable six months earlier than traditional methods.
- Novartis and Predictive Analytics: Novartis has seamlessly integrated predictive analytics and machine learning into its pharmacovigilance operations. By analyzing historical data on drug safety, they’ve achieved unprecedented accuracy in predicting potential adverse events. A research paper in “Clinical Pharmacology and Therapeutics” unveiled that Novartis’ predictive analytics reached an exceptional accuracy rate of 90%. This represents a groundbreaking level of precision and underscores the potential of predictive analytics in enhancing medication safety. Another study in the “Drug Safety” journal found that Novartis’ use of predictive analytics resulted in a 20% reduction in serious adverse events.
- AstraZeneca’s Social Media Surveillance: AstraZeneca utilizes AI to actively monitor social media platforms for mentions of their medications. This real-time monitoring has enabled them to swiftly recognize and investigate potential adverse reactions. A research study in the “Drug Safety” journal revealed that AstraZeneca’s social media monitoring achieved an 80% sensitivity and 70% specificity in identifying adverse drug reactions (ADRs). These figures represent substantial improvements compared to the sensitivity (typically around 60%) and specificity (usually around 70%) of conventional methods. Furthermore, another study published in the “Nature Medicine” journal found that AstraZeneca’s social media monitoring reduced the time for identifying ADRs by an average of 30%.
- Sanofi’s AI-Enhanced Signal Detection: Sanofi, a prominent player in the pharmaceutical sector, has seamlessly integrated AI into its signal detection processes. By analyzing electronic health records, insurance claims, and various other sources, they’ve achieved a substantial increase in the detection of previously unrecognized safety signals. Research featured in the “Pharmacoepidemiology and Drug Safety” journal disclosed that Sanofi’s AI-enhanced signal detection achieved an 85% sensitivity and 75% specificity in identifying previously unrecognized safety signals. These figures represent remarkable improvements compared to the sensitivity (typically around 50%) and specificity (usually around 60%) of conventional methods. Additionally, another study published in the “Nature Biotechnology” journal found that Sanofi’s AI-enhanced signal detection reduced the time for identifying previously unrecognized safety signals by an average of six months.
As our understanding of drug safety continues to evolve, the importance of pharmacovigilance cannot be overstated. AI-powered pharmacovigilance is not a futuristic concept; it’s a present-day reality that promises to revolutionize the landscape. With its ability to navigate the complexities of vast and diverse datasets, AI is poised to ensure that medications remain safe and effective for patients worldwide.
The Data Dynamics Advantage
In the pharmaceutical industry, the exponential growth of unstructured data presents an undeniable challenge. As companies continue to amass vast volumes of data from diverse sources, it becomes imperative to devise an effective strategy for managing and organizing this information.
Selecting the right approach for data management, whether it involves building an in-house team or partnering with a third-party vendor, can significantly impact operational efficiency and overall profitability. While addressing the expansion of unstructured data may initially seem daunting, it ultimately positions organizations ahead of competitors struggling with inefficient systems. This is precisely where Data Dynamics steps in.
Data Dynamics stands as a prominent provider of enterprise data management solutions, offering a lifeline to organizations grappling with the intricacies of unstructured data through its Unified Unstructured Data Management Platform. This comprehensive platform comprises four critical modules: Data Analytics, Mobility, Security, and Compliance.
Proven effective in over 28 Fortune 100 organizations, the Data Dynamics Platform harnesses a blend of automation, AI, ML, and blockchain technologies. It scales effortlessly to meet the demands of global enterprise workloads. With Data Dynamics at their side, enterprises can bid farewell to disparate point solutions and fragmented data perspectives. Instead, they can embrace a unified software platform that not only structures unstructured data but also unlocks invaluable data-driven insights, fortifies data security, ensures compliance and governance, and spearheads cloud data management initiatives. Ultimately, Data Dynamics envisions a future where enterprises achieve data democratization, enabling users of all technical backgrounds to instantly access, comprehend, and extract maximum insights from sprawling unstructured data landscapes.
To delve deeper into how Data Dynamics can empower your enterprise in structuring unstructured data and optimizing costs, please explore our offerings at www.datadynamicsinc.com or reach out to us at solutions@datdyn.com or (713)-491-4298.
Now that we’ve established the critical role of pharmacovigilance and the impact of AI, let’s dive deeper into what pharmacovigilance data entails and where it comes from. Here are the key components:
- Adverse Event Reports: At the heart of pharmacovigilance are adverse event reports. These reports contain information about unexpected, undesirable side effects or any other safety concerns related to a medication. They can come from various sources, including healthcare professionals, patients, and pharmaceutical companies.
- Patient Narratives: Beyond just checkboxes and medical codes, patient narratives provide a more comprehensive view of the patient’s experience with a medication. These narratives often contain valuable context and details that may not be present in structured data.
- Electronic Health Records (EHRs): EHRs are digitized records of patients’ medical history, including medications they have taken. They serve as a rich source of structured data that can be correlated with adverse event reports for deeper insights.
- Social Media and Online Forums: In an age of digital communication, patients and healthcare professionals frequently discuss their experiences with medications on social media platforms and online forums. These discussions, while unstructured, can offer early indications of potential safety concerns.
- Clinical Trials Data: Clinical trials are conducted to assess a medication’s safety and efficacy before it reaches the market. Data from these trials contribute to our understanding of a drug’s profile and potential risks.