When it comes to the energy sector, the impact of artificial intelligence (AI) is expected to be significant. While there are grand predictions about AI’s transformational potential across various domains, focusing on how it specifically manifests in the energy sector is crucial. AI holds immense promise in the energy sector as it has the potential to revolutionize the industry towards a digitized, distributed, decarbonized, and democratized energy system. However, the current reality presents a substantial gap between this vision and what is currently in place. The existing energy infrastructure, particularly in the United States, was designed for a different era and lacks the necessary real-time, granular data that is crucial for AI to fully realize its potential. Let’s take a closer look at some of the gaps, how they can be mitigated and the role of data in accelerating AI.
The Evolution of the Energy Sector
During the Transition AI conference in Boston, David Groarke, managing director of Indigo Advisory Group, presented a compelling narrative that provided a profound understanding about the energy sector and the current trends reshaping this industry. It became evident that AI is not merely a technology but a herald for a new epoch in the utility industry.
Groarke’s narrative follows a distinct arc that sheds light on the evolution of the power sector:
- Restructuring Era (1970s-1990s): This period witnessed the power sector undergoing significant restructuring, coinciding with the emergence and increasing prominence of renewable energy sources. The sector began shifting its focus towards diversifying the energy mix and exploring cleaner alternatives.
- Digitization Era (2000s-2020): From the turn of the millennium until the recent past, the energy sector experienced a phase of digitization. Advancements in technology facilitated the digital transformation of various processes and systems within the sector. This digitization served as the foundation for the energy transition, allowing for improved efficiency, data management, and the integration of renewable energy into the grid.
- Automation Era (Starting in 2020): The present era marks the advent of automation, which the power of AI has amplified. Automation, powered by AI technologies, is poised to play a pivotal role in driving the achievement of net-zero goals. It enables intelligent decision-making, optimization, and automation of energy systems, thereby facilitating the transition toward a sustainable and low-carbon future, be it enhanced operational efficiency, grid optimization, demand response, predictive maintenance, and effective integration of renewable energy sources.
Let’s dive into the fascinating world of AI and its role in transforming the energy industry.
Decoding the Era of Automation
Welcome to the automation era in the energy sector! Picture this: a bustling power plant humming with activity, but instead of relying solely on human operators, intelligent machines and AI algorithms are working hand in circuitry. This is no more a dream, but quickly becoming a reality – AI in energy and power market is projected to grow at a CAGR of 24.68% from a market valuation of US$3.103 billion in 2021 to reach US$14.527 billion in 2028.
The growth of artificial intelligence (AI) in the energy sector has been nothing short of remarkable. As energy companies strive to meet the ever-increasing demand for power while simultaneously reducing their carbon footprint, they are turning to AI as a game-changing solution. AI technologies are being integrated into every aspect of the energy sector, from exploration and production to distribution and consumption. Advanced algorithms are empowering companies to analyze vast amounts of data collected from sensors, smart meters, and other sources, providing invaluable insights into energy patterns, consumption habits, and infrastructure performance. With AI, energy companies can optimize their operations, detect anomalies, and make data-driven decisions that maximize efficiency and drive sustainable practices.
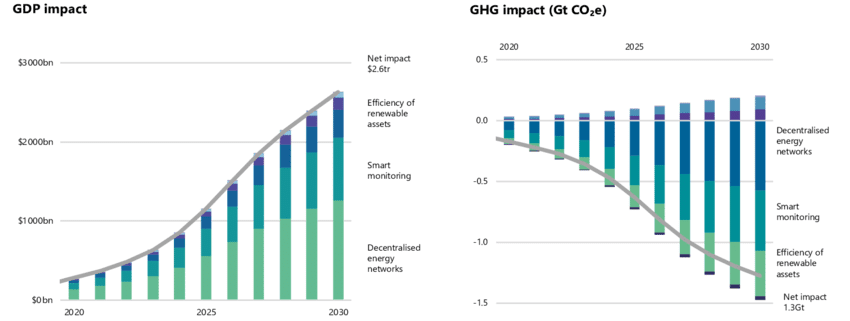
Global impact of environmental AI in the energy sector on GDP and GHG emissions in the “Expansion” scenario
Five AI-Growth Drivers
- Predictive Maintenance: AI-driven predictive maintenance is a game-changer for energy companies. By analyzing sensor data and equipment performance, AI algorithms can detect potential faults or failures before they occur. This proactive approach to maintenance can reduce downtime by up to 50% and decrease maintenance costs by 10% to 40% (according to Accenture). This means fewer unexpected disruptions and optimized maintenance schedules, leading to significant cost savings for energy companies.
- Energy Trading: AI is revolutionizing the field of energy trading. With its ability to analyze vast amounts of data and market trends in real-time, AI algorithms can optimize energy trading strategies and increase profitability. According to a report by McKinsey, AI-based energy trading algorithms have shown an average increase of 2% to 4% in trading profits. In a highly competitive market, even a slight improvement in trading performance can translate into substantial financial gains for energy companies.
- Load Forecasting: AI-powered demand response programs are proving to be effective in balancing energy supply and demand. By incentivizing consumers to adjust their energy usage during peak demand periods, energy companies can avoid costly infrastructure upgrades and reduce reliance on fossil fuel power plants. Studies by International Energy Agency (IEA) have shown that AI-based demand response programs can reduce peak electricity demand by 10% to 20%. This not only benefits the energy companies by optimizing resource allocation but also contributes to a more sustainable and resilient energy system.
- Renewable Energy Generation: AI is playing a crucial role in optimizing renewable energy generation and integration. By analyzing data from renewable sources, AI algorithms can forecast energy output, optimize power generation, and improve grid integration. According to a study published in Nature Energy, AI-driven solar power forecasting models have achieved a 30% reduction in forecasting errors compared to traditional methods. This accuracy and efficiency enable energy companies to better utilize renewable energy resources, reduce reliance on conventional power plants, and meet renewable energy targets.
- Data Generation and Anomaly Detection: Generative AI models have proven to be valuable in the generation of synthetic data that closely resembles real-world energy data. This synthetic data plays a crucial role in scenarios where obtaining real data is limited or challenging. For instance, if there is a shortage of historical energy consumption data for a specific region, a generative AI model can generate synthetic data that captures the expected patterns and characteristics of energy usage in that region. By comparing real-time data to the generated synthetic data, any deviations or anomalies can be promptly identified, enabling early detection of equipment malfunctions, grid disturbances, or cybersecurity threats.
- Digital Disruption: The integration of AI in the energy sector is indeed fostering innovation and driving the development of transformative technologies. Machine learning algorithms are revolutionizing renewable energy forecasting, leading to more accurate predictions of solar and wind power generation. According to a study published in the journal Applied Energy, the use of machine learning algorithms in solar power forecasting has resulted in a 25% reduction in forecasting errors compared to traditional methods. This improved accuracy not only enhances the grid integration of renewable sources but also enables better planning and resource optimization. Furthermore, AI-powered energy management systems are empowering consumers to take control of their energy usage. A report by the World Economic Forum highlights that smart home energy management systems, driven by AI algorithms, can reduce household energy consumption by up to 10%. By providing real-time data and insights, these systems enable consumers to make informed choices about their energy usage, identify areas of waste, and implement energy-saving measures. This not only helps individuals reduce their carbon footprint but also leads to significant cost savings.
The statistics and insights mentioned above provide a glimpse into the immense benefits that AI brings to energy companies. AI is truly a game-changer, unlocking new possibilities and driving profitability and sustainability in the energy sector. Let’s take a closer look at some real-world examples of energy companies that have reaped significant value through the adoption of AI technologies across various aspects of their operations:
- NextEra Energy: NextEra Energy, one of the largest renewable energy companies in the world, has leveraged AI to optimize their wind turbine operations. By utilizing AI algorithms to analyze real-time wind data and turbine performance, they have achieved a 20% increase in energy output from their wind farms. This improvement not only maximizes their renewable energy generation but also enhances their profitability.
- Duke Energy: Duke Energy, a leading electric power holding company in the United States, has implemented AI-based demand response programs. Through these programs, they engage customers in reducing their energy consumption during peak demand periods. Duke Energy has observed a 10% reduction in peak electricity demand as a result of these AI-driven initiatives. This enables them to better balance energy supply and demand, infrastructure optimization, and provide reliable service to their customers.
- Enel: Enel, an Italian multinational energy company, has embraced AI for predictive maintenance. By analyzing sensor data and equipment performance, they can anticipate and prevent potential failures in their power plants. This proactive approach to maintenance has reduced their downtime by up to 30% and maintenance costs by 20%. Enel’s adoption of AI-driven predictive maintenance has not only improved their operational efficiency but also reduced operational risks and improved asset lifespan.
- Tesla: Tesla, known for its innovative electric vehicles, has also ventured into the energy sector with its AI-powered energy management solutions. Through their Powerwall and Powerpack products, Tesla utilizes AI algorithms to optimize energy consumption and storage in homes and businesses. This has enabled users to reduce their reliance on the grid and maximize the utilization of renewable energy sources, leading to significant cost savings and a more sustainable energy footprint.
While the advancements in AI are undeniably impressive and indispensable in today’s world, the importance of high-quality and rich data cannot be overstated when it comes to maximizing the output and performance of artificial intelligence. Let’s unravel the significance and pivotal role of data in unlocking AI’s true potential for achieving unparalleled outcomes.
The Key to Unlocking the Power of AI lies in DATA – 7 Reasons Why
In order to fully harness the power of artificial intelligence (AI), the quality and availability of data are of paramount importance. Data serves as the fuel that powers AI algorithms, enabling them to learn, make predictions, and generate insights. However, for AI to reach its true potential, significant improvements must be made in the way we handle and utilize data. Unified data management (UDM) is the key that unlocks the untapped potential of enterprises, enabling them to capitalize on their data and unleash the full power of AI.
Here are seven key aspects in which data must improve to effectively harness the power of AI and the role of UDM:
1. Quantity and Diversity
AI systems in the energy industry thrive on large and diverse datasets. The more data available, the better AI algorithms can train and improve their accuracy.
- According to a report by Accenture, 80% of AI’s success is attributed to the quantity and quality of training data.
- A study by OpenAI found that larger and more diverse datasets significantly improve the performance and generalization of AI models.
To ensure organizations have access to substantial volumes of relevant data from various sources, such as structured databases, unstructured text, images, audio, and sensor data, unified data management comes into play. UDM enables energy companies to consolidate and integrate data from disparate sources. By bringing together data from various systems and platforms, organizations can create a comprehensive and diverse dataset for training AI models. This consolidation eliminates data silos and promotes a holistic approach to data collection, allowing AI algorithms to access a broader range of information for better accuracy and generalization in the energy industry.
2. Data Quality and Consistency
In the energy sector, the quality and consistency of data are paramount for AI algorithms. High-quality data, free from errors, noise, and biases, is crucial for training reliable models.
- IBM estimates that poor data quality costs the U.S. economy around $3.1 trillion per year.
- According to Gartner, organizations that invest in data quality management can expect a 40% reduction in operational costs and a 20% increase in revenue by 2022.
It is essential to invest in data cleaning and preprocessing techniques to eliminate inconsistencies and inaccuracies in the data. By enforcing consistent data standards and implementing data quality checks, UDM improves the overall quality of the data. This helps energy companies train AI models on high-quality data, leading to more reliable predictions and insights for tasks such as demand forecasting, grid optimization, and equipment maintenance.
3. Data Privacy and Security
As AI algorithms increasingly rely on personal and sensitive data in the energy industry, ensuring data privacy and security is of utmost importance.
- The General Data Protection Regulation (GDPR) has had a significant impact, with fines amounting to €272 million in its first year of enforcement (source: DLA Piper GDPR Data Breach Survey).
- A survey conducted by Cisco found that 83% of organizations have experienced a data breach within the past three years, emphasizing the need for robust data security measures.
Stricter regulations highlight the need for responsible data handling practices. Unified data management includes robust security measures and privacy controls. By centralizing data storage and implementing access controls, energy companies can strengthen data security. With features such as encryption, authentication, and audit trails, UDM platforms ensure data privacy compliance. This helps organizations protect sensitive information and build trust in the responsible handling of data, assuring individuals that their privacy is safeguarded.
4. Data Accessibility and Sharing
Collaboration and data sharing are crucial for AI advancement in the energy industry.
- The World Economic Forum estimates that data sharing initiatives in the energy sector could unlock $2.8 trillion in value globally by 2025.
- A study published in Nature Climate Change highlighted the importance of data sharing in renewable energy research and found that open access to energy data significantly accelerates innovation.
However, many organizations face challenges in accessing high-quality data due to proprietary restrictions, data silos, or concerns over sharing sensitive information. Unified data management facilitates efficient data sharing and collaboration. By providing a centralized platform, energy companies can simplify the process of sharing data internally and externally while maintaining control over access rights and permissions. With proper data governance mechanisms, unified data management platforms enable secure data sharing, encouraging collaboration among researchers, organizations, and AI practitioners. This collaboration leads to the accumulation of diverse datasets, fostering innovation and enhancing AI capabilities in areas such as renewable energy integration, grid management, and energy trading.
5. Ethical Considerations
To harness the power of AI ethically in the energy industry, it is vital to address bias at the data level.
- Research from the AI Now Institute revealed that over 45% of the AI and machine learning scholarly literature lacked transparency about training data sources, limiting the ability to assess biases.
Biased or skewed datasets can perpetuate discriminatory outcomes and reinforce societal inequalities. UDM platforms can incorporate ethical guidelines and frameworks. By integrating fairness and bias detection mechanisms, organizations can proactively identify biases in the data and mitigate them before training AI models. This promotes ethical AI practices and ensures that the models built upon unified data are fair and unbiased, enhancing trust and fairness in energy-related applications.
6. Continuous Data Updates
AI systems in the energy industry must be trained on up-to-date data to remain relevant and accurate over time.
- According to IDC, the global data volume is projected to reach 175 zettabytes by 2025, emphasizing the need for continuous data updates to keep AI models relevant.
- A report by Infosys revealed that 60% of organizations experience data decay of 25% or more within three years, underscoring the importance of updating data to maintain AI model accuracy.
Unified data management allows organizations to implement automated data ingestion and synchronization processes, ensuring that AI models have access to the most up-to-date data for training and inference. Real-time data integration capabilities within UDM platforms enable energy companies to continuously update their datasets, ensuring that AI models remain relevant and effective in dynamic environments where energy markets and conditions change rapidly.
7. Data Interpretability and Explainability
The “black box” nature of some AI models raises concerns regarding their interpretability and explainability.
- A survey by PwC found that 76% of executives consider the lack of transparency in AI algorithms as the biggest barrier to adopting AI technologies.
- A study published in Nature Machine Intelligence highlighted the importance of explainable AI in healthcare, showing that clinicians are more likely to trust AI models when provided with explanations for their decisions.
To gain trust and acceptance, it is crucial to develop methods that provide insights into how AI systems arrive at their decisions. Techniques like explainable AI (XAI) aim to make AI models more transparent, allowing humans to understand and verify the reasoning behind their outputs. Unified data management platforms can integrate features for data lineage and traceability. These features provide visibility into the origin and transformation of data, allowing organizations to track and explain how AI models are trained and the data they are trained on. By providing comprehensive documentation and metadata, unified data management systems enable better interpretability and explainability of AI models, improving transparency and fostering trust among users and stakeholders. This is particularly important in critical energy infrastructure systems, where clear explanations and justifications for AI-driven decisions are essential.
The Data Dynamics Advantage
Step into the future of energy transformation with the remarkable Data Dynamics unified data management platform! This award-winning software is revolutionizing the way energy enterprises harness the power of AI, empowering them to lead the charge in innovation. At the heart of this cutting-edge platform lies its ability to structure unstructured data, making sense of the vast amounts of information generated by energy companies. With its four powerful modules—Data Analytics, Mobility, Security, and Compliance—Data Dynamics delivers a comprehensive solution tailored specifically to the needs of the energy sector.
- The Data Analytics module, is designed to empower energy enterprises in uncovering valuable insights from their data. With metadata analytics as its foundation, this module dives deep into data sources, quality, and lineage, shedding light on the structure and content of information. This knowledge fuels better data discovery, integration, and governance, leading to more accurate AI model training and maximizing overall data utilization. But that’s not all. The platform goes beyond the surface by employing context analysis, delving into the meaning and relevance of the data. By considering factors like time, location, user behavior, and environmental conditions, context analytics enables AI models to make precise predictions and generate truly meaningful insights.
- Now, let’s talk about the Security module offered by Data Dynamics—a formidable defense against cyber threats. By harnessing advanced analytics, this module provides actionable insights on risk exposure, empowering organizations to securely store high-risk data with restricted access and implement intelligent file permissions. The integrity and confidentiality of sensitive information are ensured, bolstering peace of mind. Moreover, content analytics techniques unveil hidden gems within unstructured data sources, expanding the learning capabilities of AI models to encompass a wider range of data types.
- In the ever-evolving landscape of regulations and standards, the Compliance module of Data Dynamics has got you covered. Seamlessly automating data discovery and identification of relevant fields for regulatory compliance, this module utilizes industry-standard templates to classify and organize data. With the power of blockchain technology, an immutable audit log is generated to track data access activities, mitigating the risk of penalties and safeguarding the enterprise’s reputation.
- But we don’t stop there. Introducing the Mobility module, an integral part of Data Dynamics that ensures a streamlined and optimized enterprise data estate. Migrating to the cloud becomes effortless with the comprehensive migration suite, enabling seamless consolidation of storage, replication of data, and efficient management of data lifecycles. By embracing cloud adoption, Data Dynamics empowers energy enterprises to store, process, and analyze data with utmost efficiency, providing a solid foundation for AI model training and inference. Furthermore, the flexibility of the cloud fosters seamless collaboration, data sharing, and integration of AI services into existing workflows, ushering in a new era of productivity and innovation.
Data Dynamics has already proven its worth in over 26 Fortune 100 organizations, including 4 Fortune 500 energy enterprises, solidifying its reputation as a trusted solution in the energy industry. By eliminating the need for individual point solutions with fragmented data views, the platform offers a unified experience that optimizes data management tasks. The result is improved data quality, enhanced accessibility, and a cohesive infrastructure that seamlessly integrates with AI capabilities. Whether you are a small-scale energy company or a global enterprise, Data Dynamics scales effortlessly to meet your specific requirements. The platform’s fusion of automation, artificial intelligence, machine learning, and blockchain technologies ensures that you have the necessary tools to navigate the complexities of the energy landscape and drive transformational growth.
Embrace the future of energy with Data Dynamics and unlock the full potential of your data. Experience a seamless transition into the era of AI-powered efficiency and innovation, where your energy enterprise can thrive and lead the way towards a sustainable and prosperous future. To know more, visit – www.datadynamicsinc.com or contact us at solutions@datdyn.com or call us at (713)-491-4298 or +44-(20)-45520800.