Highlights:
- AI isn’t just a trend; it’s reshaping manufacturing. It’s poised to boost factory productivity by up to 30% (PwC).
- Apart from the already known use cases, there are five unconventional AI applications, like self-adaptive production lines, generative design, and AI-driven sustainability, poised to transform Industry 4.0.
- Effective data management serves as the keystone of this transformation. It extends beyond mere data storage; it’s the strategic utilization of data to extract AI’s full potential. Fueling a $20.1 billion market growth by 2026 (MarketsandMarkets), data lakes and real-time analytics are key to driving efficiency and innovation.
In this Blog:
Artificial Intelligence, or AI, is a term that has transcended its status from a buzzword to becoming a cornerstone of technological innovation across industries. While many are familiar with AI’s applications in self-driving cars, virtual assistants, and recommendation algorithms, its profound impact on manufacturing often remains in the shadows, waiting to be unveiled.
The growing impact of AI in manufacturing is not just a technological shift; it’s a revolution that is reshaping the entire industry. AI’s influence in manufacturing extends beyond the familiar narratives of automation and efficiency. One of the lesser-known but transformative aspects is its role in adaptive and sustainable manufacturing. By harnessing AI’s predictive capabilities and real-time data analysis, manufacturers can dynamically adjust their production processes to minimize waste and resource consumption. This real-time adaptability is particularly valuable for industries with variable demand or complex manufacturing environments. AI can optimize the entire production ecosystem, from raw material usage to energy consumption, reducing environmental impact and lowering operational costs. Forward-thinking companies are already leveraging AI to create “smart factories” that respond to changing conditions, reducing waste and enhancing their sustainability profiles. Lets look at 5 less-talked-about facets of AI in manufacturing. They represent a paradigm shift in how products are designed, produced, and maintained, ushering in an era of smart, efficient, and sustainable manufacturing.
5 Lesser Known Applications of AI in Manufacturing
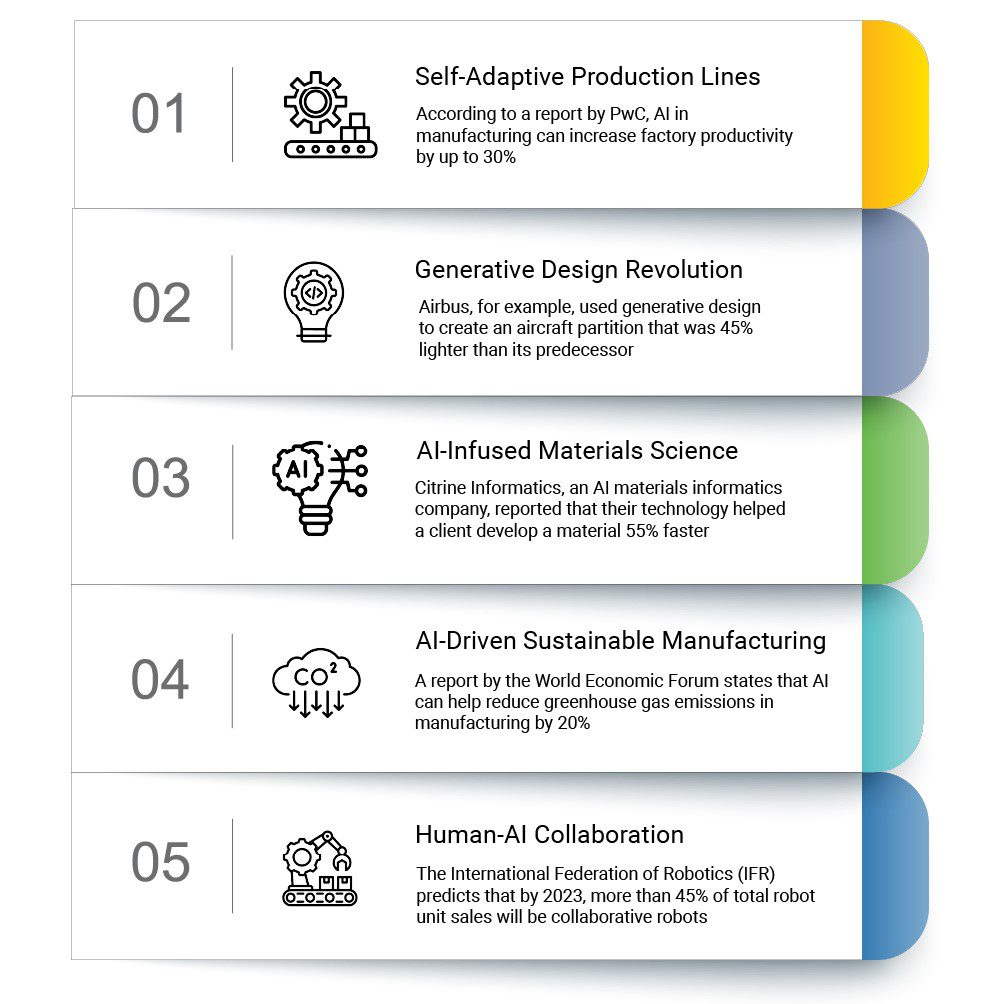
- Self-Adaptive Production Lines: AI’s role in manufacturing extends far beyond automation; it’s now about self-adaptive production lines. Imagine a factory where machines can independently adapt to changing production requirements. AI algorithms analyze real-time data to optimize the entire manufacturing process, adjusting parameters, schedules, and even maintenance needs on the fly. This level of flexibility and responsiveness is reshaping the way factories operate, making them highly efficient and adaptive. According to a report by PwC, AI in manufacturing can increase factory productivity by up to 30%.
- Generative Design Revolution: Generative design, powered by AI, is redefining how products are conceived and engineered. Instead of relying on human designers, generative design tools use algorithms to explore countless design possibilities based on defined parameters. This results in incredibly efficient, lightweight, and high-performance designs that human designers might have never conceived. Airbus, for example, used generative design to create an aircraft partition that was 45% lighter than its predecessor.
- AI-Infused Materials Science: The development of new materials has always been a critical aspect of manufacturing. AI is now accelerating this process by simulating and predicting the properties of materials at a molecular level. This allows for the creation of materials with highly specific characteristics, opening doors to innovative products in industries like aerospace, electronics, and healthcare. Citrine Informatics, an AI materials informatics company, reported that their technology helped a client develop a material 55% faster.
- AI-Driven Sustainable Manufacturing: Sustainability is a pressing concern, and AI is a powerful tool for addressing it. AI algorithms are optimizing manufacturing processes to reduce energy consumption, minimize waste, and enhance recycling. These algorithms also help in the development of sustainable materials and products. Think of it as a new era of “green” manufacturing. A report by the World Economic Forum states that AI can help reduce greenhouse gas emissions in manufacturing by 20%.
- Human-AI Collaboration: While AI is automating many tasks, it’s also enhancing the capabilities of human workers. Collaborative robots, or “cobots,” work alongside humans on the factory floor, taking care of the dull, dirty, or dangerous tasks, while humans focus on complex decision-making and creative aspects of production. The International Federation of Robotics (IFR) predicts that by 2023, more than 45% of total robot unit sales will be collaborative robots.
The Unseen Impact of Data in AI-Driven Manufacturing
It is clearly understood that in Industry 4.0, AI has emerged as the linchpin that propels the manufacturing sector toward greater efficiency, innovation, and competitiveness. Yet, the key to unlocking the full potential of AI in manufacturing lies not just in its adoption but in how effectively data is managed within this paradigm.
In this age of AI-driven manufacturing, data is the lifeblood that fuels the intelligence behind the machines. The digital exhaust from every production process, the intricate nuances of equipment performance, and the avalanche of sensor data all hold the promise of insights that can enhance decision-making, optimize operations, and elevate quality. However, to capitalize on AI’s transformative capabilities, manufacturing enterprises must navigate the complexities of data management with precision. Efficient data management is the bridge that links AI’s immense potential to tangible outcomes, making it a cornerstone of modern manufacturing success. It is not just about collecting and storing information; it’s about conducting a grand orchestra of data elements, transforming raw bytes into actionable insights, and orchestrating the manufacturing ballet with precision.
However, what exactly does “Effective Data Management” entail within the domain of AI-driven Manufacturing? Even more crucial, what tangible business value does it deliver? Delve into these 10 key drivers for insights.
- Data Lakes and Edge Computing: Effective data management involves the creation of data lakes that house diverse datasets. These data lakes are powered by scalable distributed file systems, enabling the processing of petabytes of data close to the edge. The data lakes market is expected to grow from $7.9 billion in 2021 to $20.1 billion by 2026, at a CAGR of 20.5% (MarketsandMarkets).
- Machine Learning Models for Data Cleansing: AI-based data management deploys machine learning models like autoencoders and convolutional neural networks (CNNs) to automatically clean data. These models utilize deep learning techniques to detect anomalies and patterns within data. In the data cleaning market, the automated data cleaning segment is expected to grow at a CAGR of 14.4% from 2021 to 2028 (Grand View Research).
- Data Ingestion Pipelines: In a technical context, data management encompasses the creation of data ingestion pipelines using technologies like Apache NiFi. These pipelines efficiently collect data from various sources while adhering to data governance policies. Data pipeline orchestration is predicted to become a $1.75 billion market by 2027, with a CAGR of 10.7% (MarketsandMarkets).
- IoT Sensor Integration: Effective data management integrates data from a multitude of IoT sensors. This involves configuring these sensors to transmit data in real-time, which is then processed by AI algorithms to offer insights into machine performance and predictive maintenance. The global predictive maintenance market is projected to reach $14.7 billion by 2026 at a CAGR of 24.2% (MarketsandMarkets).
- Unstructured Data Processing with NLP: Managing unstructured data requires Natural Language Processing (NLP) algorithms that utilize recurrent neural networks (RNNs) and transformer models. These models enable machines to comprehend human language and extract insights from textual data. Data Dynamics Analytics module, powered by a Data Science Engine, AI/ML, and NLP helps in accurate discovery, classification, and tagging for data lifecycle management, sensitive data protection and usage pattern analysis. NLP technology is expected to reach a market size of $43.3 billion by 2025, growing at a CAGR of 21.0% (MarketsandMarkets).
- Supervised Learning for Predictive Maintenance: Data management involves the utilization of supervised learning algorithms like decision trees and support vector machines for predictive maintenance. These algorithms use historical data to predict machine failures and recommend proactive maintenance schedules. The predictive maintenance market is forecasted to reach $12.2 billion by 2023, growing at a CAGR of 25.2% (MarketsandMarkets).
- Data Encryption and Blockchain: Effective data management involves robust encryption algorithms such as Advanced Encryption Standard (AES) for data at rest and in transit. Additionally, blockchain technology ensures data security and immutability. The blockchain technology market is projected to reach $39.7 billion by 2025, with a CAGR of 67.3% from 2020 to 2025 (MarketsandMarkets).
- Real-time Data Analytics: Data is processed and analyzed in real-time through complex data analytics tools. This includes the utilization of data analytics technologies like Data Dynamics for metadata, content and context analytics. The global big data analytics market is estimated to grow from $138.9 billion in 2020 to $229.4 billion by 2025, at a CAGR of 10.6% (MarketsandMarkets).
- AI-Driven Quality Control: Technical data management integrates AI into quality control processes. Computer vision systems powered by convolutional neural networks (CNNs) and recurrent neural networks (RNNs) analyze product images for defects, while machine learning models identify quality issues in real-time. The computer vision market is forecasted to reach $17.4 billion by 2023, with a CAGR of 8.9% from 2018 to 2023 (MarketsandMarkets).
- Advanced Data Storage Solutions: Effective data management leverages advanced data storage solutions such as NoSQL databases like MongoDB and Cassandra for handling massive data volumes efficiently. These databases offer the scalability required in the era of AI-driven manufacturing. The NoSQL market is anticipated to reach $22.3 billion by 2026, growing at a CAGR of 22.3% from 2021 to 2026 (MarketsandMarkets).
AI’s growing impact on manufacturing is fundamentally altering the way products are designed, produced, and delivered. From predictive maintenance to supply chain optimization and personalized manufacturing, AI-driven applications are reshaping the industry. The uncharted territory of AI in manufacturing holds immense potential, with data management at its core. As we venture further into this exciting landscape, the innovative use cases and the critical role of data will continue to guide us toward unprecedented levels of efficiency, quality, and customization. In this age of digital transformation, the manufacturing sector is poised for a renaissance that few could have imagined, and AI is the key to unlocking its full potential.
The Data Dynamics Advantage
Data Dynamics’ Unified Data Management Platform offers a pioneering solution to manufacturing companies seeking to harness the full potential of their data in the journey toward Industry 4.0 and AI applications. By efficiently structuring unstructured data, this platform acts as the cornerstone for informed decision-making. Its robust analytics capabilities uncover invaluable insights from the data, empowering manufacturing firms to make data-driven choices that enhance operational efficiency. With data that is now easily accessible, companies can leverage AI models, such as predictive maintenance and real-time supply chain optimization, more effectively. In essence, Data Dynamics’ Unified Data Management Platform lays the foundation for AI’s transformative role in Industry 4.0 by providing manufacturers with the structured, analyzed, and accessible data necessary to reshape their processes, boost agility, and drive unparalleled efficiency in this rapidly evolving industrial landscape.
- Streamlined Data Management: Utilize a unified platform to effortlessly integrate compliance, security, and unstructured data management, creating a holistic AI journey.
- Proactive Risk Mitigation: Identify potential risks within unstructured content early on, empowering proactive risk management and compliance adherence.
- Protection of Sensitive Data: Employ a combination of AI/ML-powered content analysis and a Data Science Engine to reliably detect and address PII and sensitive data.
- The 4S Data Approach: Embrace a Simplified, Secure, Strict, and Smart approach to extract invaluable insights from enterprise data in a secure, regulated, and optimized manner.
- Comprehensive Data Governance: Attain deep data visibility, stringent compliance adherence, and unchangeable audit reporting through blockchain for optimal data governance and informed decision-making.
- Intelligent Data Organization: Facilitate intelligent data organization by using advanced file identification and categorization, secure storage, compliance classification, seamless data migration, and reporting, driving a complete data transformation.
To dive deeper into the realm of possibilities that Data Dynamics can unlock for your enterprise and ensure that your business maintains its pioneering edge, visit us at www.datadynamicsinc.com. You can also reach out to us through email at solutions@datdyn.com or call us at (713)-491-4298. We’re here to assist you on your journey towards data excellence!