- During the pandemic, AI swiftly repurposed drugs like baricitinib for COVID-19, saving time and ensuring rapid treatment access. AI predicts compound interactions, reducing drug discovery timelines and costs significantly.
- Despite its success within some organizations, widespread adoption faces hurdles from cumbersome pharmaceutical practices, prompting an exploration of the challenges existing in the industry.
- Delving into the intricacies of the pharmaceutical landscape has revealed eight formidable challenges confronting enterprises today. Yet, with the transformative power of AI, these challenges can now be effectively addressed.
- However, it’s crucial for enterprises to understand that data is the lifeblood of AI, necessitating meticulous management and strategic utilization. Within this context, Ethical Data Management and Data Democratization stand out as pivotal pillars, laying the groundwork for responsible AI advancement.
- Acting as a central nexus, Unified Data Management (UDM) emerges as the linchpin, seamlessly integrating both Ethical Data Management and Data Democratization and steering the industry towards innovation driven by collective intelligence and a commitment to enhancing patient outcomes.
The urgent demand for effective treatments became undeniable during the pandemic’s grip on the world. However, the traditional route for developing drugs is known for its lengthy and costly process. That is when AI stepped in as a beacon of hope. By sifting through vast amounts of medical data, AI algorithms could pinpoint drugs with established safety records that could combat COVID-19. A notable instance occurred at AstraZeneca, where AI aided in identifying baricitinib, a medication for rheumatoid arthritis, as a potential remedy for COVID-19 patients. This swift repurposing not only saved crucial time but also tapped into existing manufacturing and distribution networks, ensuring speedy access to the drug for patients in need. Although this breakthrough occurred within one organization and led to a breakthrough, its widespread adoption across the industry was prevented due to cumbersome pharmaceutical practices. So, what are the formidable challenges pharmaceutical companies grapple with? How can AI help these companies navigate these obstacles? Let’s deep dive into this.
Navigating the Pharma Landscape: Tackling the Eight Biggest Challenges with AI-Powered Solutions
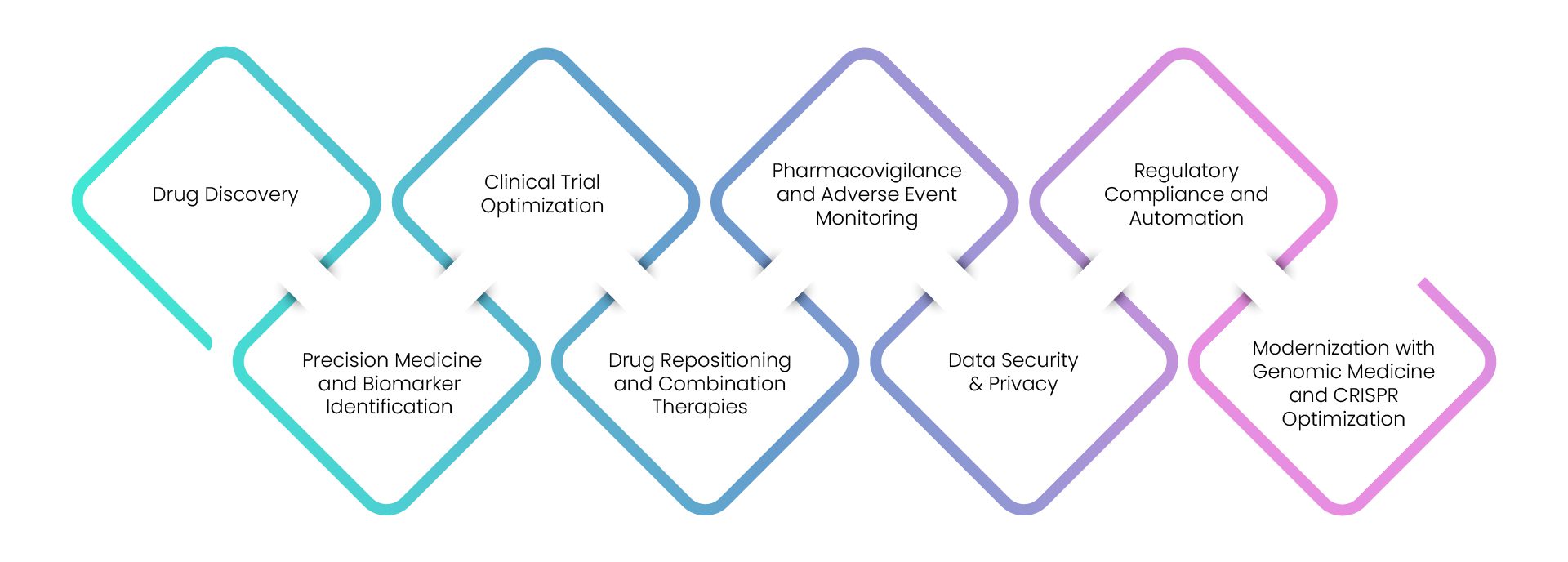
Drug Discovery
Traditional drug discovery methods have long grappled with the challenges of protracted timelines and escalating costs. The conventional trial-and-error approach, reliant on manual analyses and experimentation, often results in delays in identifying viable drug candidates. The intricacies of biological interactions pose formidable obstacles. The pharmaceutical industry has been hindered by the inability of traditional methods to efficiently predict compound interactions with specific biological targets. This lack of efficiency significantly contributes to the prolonged timeline of drug discovery. According to a report by Deloitte, the average cost of developing a new drug exceeds $2.5 billion, with timelines extending over a decade. In contrast, AI algorithms have demonstrated remarkable efficiency.
In stark contrast, AI revolutionizes this landscape by predicting compound interactions with biological targets. Quantum machine learning algorithms analyze vast datasets, significantly accelerating the identification of potential drug candidates or repurposing existing drugs. This streamlined approach not only reduces time and costs but also outperforms traditional methods by remarkable margins, as evidenced by AI algorithms analyzing 100 million molecules in seconds to identify a cancer drug candidate that surpassed traditional approaches by 100x.
Precision Medicine and Biomarker Identification
The complexity of analyzing diverse patient data, particularly genomic information, presents a significant challenge for traditional methods in precision medicine. Conventional approaches struggle to efficiently unravel complex disease pathways and identify relevant biomarkers. The siloed nature of data sources and the time-consuming manual processes involved hinder the seamless integration of diverse patient data. Moreover, privacy concerns and ethical considerations surrounding the use of patient data have been longstanding challenges, impeding the progress of precision medicine.
AI’s prowess lies in analyzing diverse patient data, unraveling complex disease pathways, and identifying relevant biomarkers. According to a study by Stanford Medicine, AI analysis of 1 million patient records uncovered a genetic variant linked to increased response to a specific diabetes medication, enabling personalized treatment for 20% of patients. By delving into genomics, proteomics, and clinical records, AI facilitates the identification of patient subgroups that respond uniquely to treatments. This enables the development of tailored therapies, optimizing treatment efficacy while minimizing adverse effects based on individual genetic profiles and disease characteristics.
Clinical Trial Optimization
Traditional clinical trial models face substantial challenges, particularly in the areas of patient recruitment and real-time monitoring. Identifying suitable participants is often a time-consuming and labor-intensive process, leading to delays and increased costs. The conventional model’s inefficiencies in optimizing trial protocols and predicting potential risks contribute to prolonged timelines and escalating expenses. Ethical considerations regarding the use of AI algorithms for decision-making in clinical trials further complicate the adoption of more efficient processes.
AI’s predictive analytics and machine learning algorithms play a pivotal role in optimizing these trials, reducing patient recruitment time, and bringing drugs to market faster. These technologies revolutionize patient recruitment by discerning intricate patterns within diverse datasets and optimizing candidate selection. Moreover, AI’s intricacies extend to protocol optimization and risk prediction, where advanced analytics assess multifaceted variables, addressing challenges associated with the multidimensional nature of trial data. Real-time monitoring, another feat, involves continuous analysis of diverse, real-time data streams, enabling adaptive trial designs and dynamic adjustments to parameters. The convergence of these capabilities accelerates trial timelines, enhances success rates, and fundamentally reshapes traditional trial methodologies. GNS Healthcare reported a 50% reduction in patient recruitment time for a rare disease trial, resulting in the drug reaching the market two years faster.
Drug Repositioning and Combination Therapies
The traditional approach to drug development, involving the creation of new compounds, grapples with prolonged timelines and high costs. The challenges lie in validating predictions made by traditional methods for repurposing existing drugs in real-world scenarios. Ensuring the safety and efficacy of repurposed drugs presents significant hurdles, and the identification of optimal combinations of medications involves complexities in understanding potential synergies and addressing possible adverse effects.
AI algorithms analyze extensive biomedical databases to identify opportunities for drug repositioning—finding new therapeutic uses for existing drugs. These algorithms also explore synergistic effects between different medications, suggesting combinations that could potentially enhance treatment efficacy against complex diseases, thereby reducing the need for de novo drug development. According to Biogen, AI analysis of medical records identified a repurposed drug for Alzheimer’s disease with a 70% success rate in Phase II trials.
Pharmacovigilance and Adverse Event Monitoring
Conventional pharmacovigilance heavily relies on reactive measures, with a reliance on post-market reports to identify adverse events. This reactive approach proves inadequate in the face of the vast and unstructured nature of real-world patient data. Traditional methods struggle to efficiently detect potential adverse events and differentiate between true signals and background noise. Moreover, ethical considerations emerge in utilizing data from unconventional sources such as social media, adding to the challenges faced by traditional pharmacovigilance.
AI, with its natural language processing (NLP) algorithms, takes a proactive stance by sifting through diverse sources of real-world patient data, including social media, electronic health records, and clinical notes, to detect potential adverse events associated with specific medications. This proactive approach to pharmacovigilance enables early identification of safety concerns, facilitating timely interventions and ensuring patient safety post-market. Roche reported NLP analysis of social media data detected a rare side effect of a new drug 2 weeks before official reporting, potentially preventing further harm.
Data Security & Privacy
The escalating frequency of cyberattacks poses a significant challenge to pharmaceutical companies relying on traditional cybersecurity methods. The industry’s digitization amplifies the vulnerability of valuable customer data to security breaches. Traditional security measures struggle to adapt to the evolving threat landscape, and the challenge lies in balancing the need for advanced threat detection with stringent privacy concerns. For instance, the pharmaceutical industry experienced a 25% increase in cyberattacks in 2023 compared to 2022. This means that, on average, a pharmaceutical company was attacked every 6 minutes. Also, the cost of a data breach in the pharmaceutical industry is one of the highest of any industry. In 2023, the average cost of a data breach in the pharmaceutical industry was $4.82 million.
In this age where data protection is paramount, AI takes center stage in safeguarding customer Personally Identifiable Information (PII) and sensitive data. As pharmaceutical operations digitize, AI-powered solutions become the vanguard of advanced threat detection, encryption, and access controls. These technologies swiftly identify anomalies, fortifying the industry’s defenses against cyber threats. AI-driven authentication methods, including biometrics and behavioral analysis, contribute to robust access controls, aligning with stringent regulatory compliance requirements. This ensures not only fortified data security but also accurate and compliant documentation, expediting regulatory approvals.
Regulatory Compliance and Automation
Traditional regulatory compliance processes are marred by manual reviews, leading to delays and potential errors. The industry’s resistance to change and the need for retraining personnel to work alongside automated systems pose significant challenges. Moreover, ensuring transparency and interpretability in the decision-making processes of AI-driven systems becomes crucial for meeting regulatory standards and ethical considerations. Striking a balance between traditional manual reviews and the incorporation of AI automation adds a layer of complexity to regulatory compliance.
AI streamlines regulatory compliance by automating documentation processes, ensuring accuracy, and expediting approvals. Moreover, the implementation of explainable AI (XAI) mechanisms further elevates this paradigm, offering transparency into decision-making processes. Through advanced algorithms and machine learning, it not only navigates the complexities of compliance requirements but also provides a clear and understandable rationale for its decisions. This expedites the regulatory approval process and ensures alignment with ethical considerations, providing a robust framework for compliance in the rapidly evolving landscape of the pharmaceutical industry. An AI system automated 80% of regulatory document reviews, reducing approval time by 3 months and freeing up human reviewers for complex tasks.
Modernization with Genomic Medicine and CRISPR Optimization
The challenges in understanding diseases at a molecular level through genomic medicine are accentuated by the vast complexities of genetic data. Traditional methods grapple with the sheer volume and intricacies of genomic information. Additionally, ethical considerations related to gene editing, especially in human trials, present significant challenges. The balance between the potential benefits of optimizing CRISPR-based gene editing techniques and ethical implications remains an ongoing challenge in the field of genomic medicine, hampering the progression toward more efficient and targeted therapies.
AI’s prowess in analyzing genetic data aids in understanding diseases at a molecular level, facilitating the development of targeted therapies. According to the University of California, AI analysis of genomic data identified gene mutations contributing to ALS, leading to the development of a targeted therapy with 60% improvement in motor function in preclinical models. Additionally, AI assists in optimizing CRISPR-based gene editing techniques, enhancing precision and efficacy in gene therapy approaches.
The traditional drug discovery process typically takes around ten years to bring a single drug to market due to its intricate and time-consuming nature. It involves various stages, from initial research to clinical trials and regulatory approvals. However, as discussed, the urgency brought about by the COVID-19 pandemic spurred a paradigm shift in the pharmaceutical industry. AI swiftly expedited the drug discovery process, leveraging machine learning algorithms to analyze vast amounts of data and identify potential drug candidates. This accelerated pace was evident in initiatives to repurpose existing drugs, identify novel compounds, and streamline clinical trials for COVID-19 treatments and vaccines.
The Bedrock of AI: DATA
In the dynamic landscape of pharmaceuticals, the relationship between Artificial Intelligence (AI) and data emerges as an unequivocal catalyst, laying the robust foundation for innovation and progress. Far beyond a conventional resource, data in this context is akin to the very DNA that propels advancements in research and development and, ultimately, elevates patient care within the industry. Its significance resonates profoundly, acting as the driving force behind predictive analytics, expediting drug discovery, and refining the intricate landscape of personalized medicine. In essence, data serves as the indispensable bedrock upon which the edifice of AI in pharma is erected.
This inseparable connection between AI and data leads us to the paramount focuses for responsible AI advancement: Ethical Data Management and Data Democratization. Ethical Data Management demands a meticulous and transparent approach to the collection, storage, and utilization of data. This underscores the establishment of robust data governance frameworks, ensuring compliance with privacy regulations and fortifying the sanctity of patient information. In an industry where precision and integrity are non-negotiable, the ethical handling of data becomes a linchpin, directly influencing patient trust and regulatory adherence.
Complementary to this ethical imperative is the concept of Data Democratization, which aims to dismantle traditional barriers by making data accessible and comprehensible across diverse stakeholders in the pharmaceutical ecosystem. The imperative here is fostering collaboration among researchers, clinicians, and data scientists to harness the collective intelligence embedded in diverse datasets. By democratizing data, insights are liberated from silos, cultivating a collaborative and innovative environment that permeates the entire industry.
Unified Data Management (UDM) emerges as the keystone, seamlessly integrating both Ethical Data Management and Data Democratization. Functioning as a central hub, UDM not only streamlines data from disparate sources, ensuring quality, consistency, and security, but also facilitates responsible data sharing in alignment with ethical standards. This convergence of ethical principles and technological efficiency makes UDM the enabler in the responsible deployment of AI. Furthermore, UDM systematically breaks down data silos, promoting seamless collaboration among stakeholders and democratizing insights for a more inclusive and transparent pharmaceutical ecosystem.
As the pharmaceutical industry embarks on a transformative journey fueled by AI, the role of data as the lifeblood cannot be overstated. Ethical Data Management and Data Democratization are the twin pillars supporting responsible AI advancements. Unified Data Management serves as a bridge, transcending technological challenges and ethical considerations, propelling the industry toward a future where innovation is driven by collective intelligence, ethics, and a commitment to improving patient outcomes.
The Data Dynamics Advantage
Data Dynamics stands as the unrivaled leader in enterprise data management solutions, spearheading the charge in orchestrating Data Democratization within an AI-driven. At the core of our offerings lies the prestigious Unified Data Management (UDM) Software, meticulously designed to empower organizations in structuring unstructured data and extracting unparalleled insights securely, governed, and optimized. Battle-tested in 300+ organizations, including 28 Fortune 100 entities, it dynamically adapts to evolving macroeconomic conditions and the fluid data landscape through its four modules: Data Analytics, Mobility, Security, and Compliance. Fueled by a strategic fusion of automation, AI, ML, and blockchain, our software is the cornerstone solution for navigating modern data challenges.
Functioning as the central nervous system of enterprise data, the UDM Software seamlessly integrates Data Security, Governance, and Intelligence within the guardrails of IT infrastructure. The paramount advantage of our software lies in liberating enterprises from fragmented, point solutions and disjointed data perspectives. With our UDM software, businesses benefit from a holistic approach that structures unstructured data, unlocks transformative insights, fortifies data security, ensures compliance and governance, and propels efficient cloud data management.
At Data Dynamics, Data Democratization is our commitment to an enterprise where every individual—whether a consumer, employee, or stakeholder—has the right to control their data destiny. This commitment is about empowering individuals with the knowledge to drive meaningful change. Privacy and security aren’t mere features for us; they are fundamental rights woven into the fabric of our solutions. Additionally, we advocate for ethical data management practices. Responsible data handling, citizen data rights, and data custodianship are not just words; they are integral to our ethos. We uphold the principles of responsible data management, ensuring that our customers are empowered with solutions ushering in the next generation of data management—one that is built on respecting the rights of individuals over their data, imparting maximum control, and embracing the role of responsible custodians in the data ecosystem.
With Data Dynamics, you’re not just managing data; you’re harnessing its true potential while ensuring ethical AI usage. To know more, visit www.datadynamicsinc.com or reach out to us at solutions@datdyn.com or (713)-491-4298.