Highlights:
- AI, once a futuristic concept, now seamlessly influences our daily lives, from digital assistants to personalized ads, urging us to explore its ethical implications.
- While AI brings remarkable progress, it has an obscured ethical underbelly. Discrimination, Privacy concerns, sensitive data disclosure, amplification of bias, quality and access disparities challenge its ethical dimensions.
- Ethical AI hinges on structured data management. It ensures reliability, transparency, and fairness, addressing issues from bias mitigation to data privacy protection.
- Some organizations, such as JPMorgan Chase, the Mayo Clinic, Enel, Siemens, and GlaxoSmithKline, exemplify mindful AI adoption, respecting ethics across various industries.
In the Blog:
Artificial Intelligence (AI), once a futuristic concept, has quietly woven itself into the fabric of our daily lives. Its pervasive influence is evident in the digital assistant that suggests our daily routines, the personalized ads that follow us online, and even the recommendations that influence our choices in music, movies, and books. While we embrace the conveniences AI offers, it’s crucial to recognize the profound impact it has on our lives, even in ways we may not readily perceive.
As AI continues to advance, it becomes increasingly imperative to shed light on unseen ethical dimensions. The power to innovate responsibly and ensuring AI works to the benefit of all lies in our collective ability to recognize and address these ethical concerns. By doing so, we can shape a future where AI elevates our lives without compromising our values and principles.
The Dark Side of AI
While the advancement of Artificial Intelligence (AI) has brought about remarkable progress and innovation, it’s essential to acknowledge that AI, like any powerful tool, also has a darker side. Beyond the dazzling capabilities and conveniences it offers, AI is entangled with a web of ethical dilemmas and consequences. Let’s take a look at how it’s affecting some of the biggest industries.
- Finance: Discriminatory Financial AI
Unethical AI usage in finance often results in discriminatory lending practices. AI algorithms, which rely on historical data for decision-making, can inadvertently perpetuate biases based on race, gender, age, or socioeconomic factors. This can lead to discriminatory loan approvals, rejections, and interest rates. Consumers from underrepresented groups may find themselves at a disadvantage, struggling to access loans on fair terms. The hidden nature of these biases within AI systems not only erodes trust in financial institutions but also conceals the sources of these disparities, making it difficult for affected individuals to seek recourse. In the absence of awareness and ethical regulation, systemic financial inequalities persist, undermining societal progress.
- Healthcare: Patient Privacy Breaches
Ethical concerns in healthcare AI often revolve around patient privacy. Unethical AI usage may lead to data breaches or unauthorized access to sensitive medical information. Patient records, diagnostic results, and medical histories, often containing highly personal information, are at risk. When this information is exposed or misused, it can lead to stigmatization, discrimination, and a loss of trust in healthcare providers. Patients may become hesitant to share critical health information, impairing the quality of care they receive. The hidden risks associated with patient data misuse, combined with the lack of transparency in AI systems, intensify the ethical challenges in healthcare.
- Manufacturing: Product Quality and Safety
Unethical AI usage in manufacturing can have far-reaching consequences for consumers, particularly in terms of product quality and safety. If AI-driven quality control systems inherit biases or are manipulated to cut costs, consumers may unknowingly purchase substandard or unsafe products. The consequences can range from inconvenience and financial loss to severe safety hazards and health risks. Trust in the manufacturing sector diminishes, and safety concerns often remain obscured until accidents or incidents occur. The hidden ethical risks in AI-driven manufacturing processes emphasize the need for vigilance, transparency, and ethical regulation to protect consumers from suboptimal and unsafe products.
- Energy: Energy Affordability and Access Disparities
In the energy sector, unethical AI usage can lead to disparities in energy affordability and access. AI systems, when designed to prioritize profit over ethical considerations, can implement dynamic pricing models that disproportionately burden lower-income consumers. This can result in energy becoming unaffordable for vulnerable populations, affecting their quality of life and exacerbating existing socioeconomic disparities. Additionally, unethical AI deployment in energy grids can lead to unreliable energy supply and energy shortages, with marginalized communities bearing the brunt of the impact. Consumer understanding of how AI decisions influence energy access and affordability is often limited, highlighting the importance of raising awareness and advocating for ethical practices in the energy sector to ensure equitable access to energy resources.
Ethical Considerations in the Development of AI and The Role of Data
Ethical AI development demands vigilance. The foundation lies in data – the lifeblood of AI. Proper data handling and ethical sourcing are prerequisites to responsible AI. The necessity for transparency, accountability, and fairness in AI development cannot be overstated. The power to shape the ethical landscape of AI rests not only in the hands of developers but also in those who contribute data. According to a study by Stanford University, 75% of AI and machine learning applications do not perform as expected due to poor quality data, highlighting the importance of data in AI success. Unified Data Management (UDM) plays a pivotal role in ensuring that data is managed in a secure and ethical manner, thereby enhancing the overall trustworthiness and reliability of AI systems. Let’s discover how.
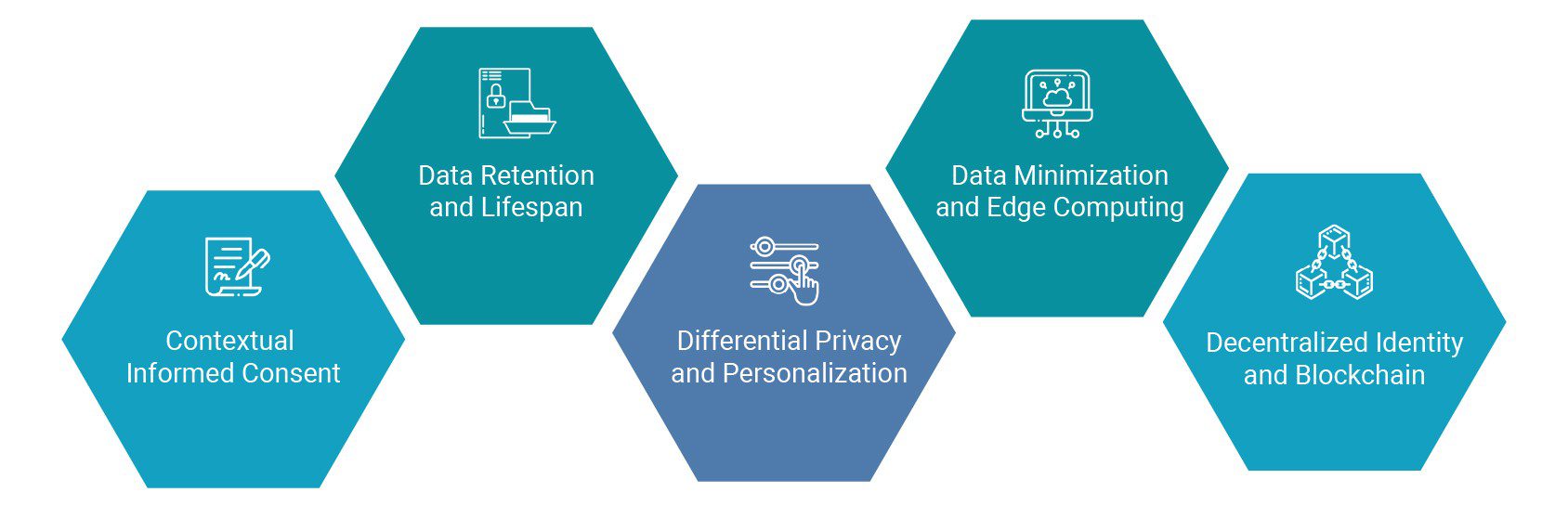
- Contextual Informed Consent:
In the era of AI, obtaining informed consent remains a critical issue. However, ensuring that users are not only aware of how their data is used but also the context in which it is employed is essential. Innovative approaches may involve creating dynamic consent systems that adapt to different data usage scenarios. These systems could allow users to specify the extent and limits of data usage for specific AI applications, providing a granular level of control over their personal information. According to a study by Cisco, 42% of consumers are willing to share their data with companies they trust, highlighting the importance of transparent consent mechanisms
UDM can play a pivotal role in implementing dynamic consent systems. AI developers can create a centralized platform where users can manage their consent preferences across various AI applications. This ensures that individuals have a comprehensive view of how their data is used and enables them to set context-specific permissions for different AI use cases.
- Data Retention and Lifespan:
AI developers should consider the ethical implications of data retention. Beyond user consent, data lifespan should be part of the conversation. Innovative approaches may involve developing self-destructing data after a predefined period or when it’s no longer necessary for the intended AI application. This approach ensures that data doesn’t persist indefinitely, minimizing the risk of unintended or unauthorized use.
UDM allows for effective data governance. AI developers, with the help of UDM, can set data retention policies and automate data lifecycle management. This ensures that data is automatically deleted or anonymized as per predefined rules, reducing the risk of data breaches and unauthorized access while maintaining compliance with privacy regulations.
- Differential Privacy and Personalization:
Striking the right balance between personalization and privacy is a complex challenge. AI developers must innovate by employing differential privacy techniques to protect individual data while still delivering personalized experiences. This approach adds noise or randomness to the data before processing, making it harder to identify individuals while preserving the overall utility of the dataset for AI models. A study by Apple revealed that differential privacy was used in the analysis of user data, ensuring privacy while still delivering personalized experiences.
UDM can centralize data processing while preserving user privacy. It enables the aggregation of data at a central point, applying differential privacy techniques to protect individual records. This approach provides the benefit of personalization while minimizing the risk of reidentification, as UDM ensures that sensitive data remains confidential even during analysis.
- Data Minimization and Edge Computing
Edge computing, where AI processes data locally on devices, can help minimize data exposure to external servers. AI developers should consider moving away from the practice of over-collecting data and focus on processing data at the source, reducing the need to transfer sensitive information to the cloud. This minimizes data privacy risks and fosters a more secure ecosystem for AI.
UDM can facilitate efficient data minimization. By using UDM solutions, developers can implement data minimization strategies and anonymization techniques at the edge. UDM ensures that only necessary data is processed and transferred, reducing the exposure of sensitive information while maintaining data integrity.
- Decentralized Identity and Blockchain
Exploring the use of blockchain technology for decentralized identity management can be an innovative way to protect data privacy. Users can have more control over their identity and personal data, and AI applications can access relevant information securely and with explicit permission. Decentralized identity solutions can also foster trust in the AI ecosystem, as users have visibility into data access and usage.
UDM can help maintain decentralized identity records securely. By integrating UDM with blockchain technology, developers can ensure the secure and decentralized management of user identities and permissions. UDM with blockchain can provide a comprehensive audit trail, allowing users to track and control how their identity and data are used across AI applications.
Let’s now check out five organizations that have demonstrated mindfulness of ethical considerations while using AI across different industries:
- Finance: JPMorgan Chase, a prominent financial institution, has shown commitment to AI ethics. The company has developed AI algorithms that assess loan applicants for creditworthiness. JPMorgan Chase is proactively addressing potential bias and discrimination issues by investing in AI fairness and transparency tools to ensure that lending decisions are equitable.
- Healthcare: The Mayo Clinic, a renowned healthcare organization, has been a leader in ethical AI use. They employ AI in healthcare for diagnosis and treatment recommendations while prioritizing patient privacy. Mayo Clinic has implemented robust data security and privacy measures to protect sensitive medical information, ensuring ethical data handling.
- Energy: Enel, a global energy company, is committed to ethical AI usage in the energy sector. The company uses AI to optimize energy distribution through smart grids while respecting consumer data privacy. Enel has taken steps to secure consumer data, protect against breaches, and provide transparency about data handling practices.
- Manufacturing: Siemens, a multinational manufacturing and engineering company, embraces AI in its manufacturing processes. Siemens is known for its commitment to ethical considerations related to workforce transformation. The company focuses on retraining and upskilling employees to address potential job displacement caused by automation and AI technologies.
- Pharmaceuticals: GlaxoSmithKline, a global pharmaceutical company, uses AI for drug discovery. GSK emphasizes ethical AI in the pharmaceutical sector, particularly in the context of intellectual property. The company has engaged in discussions and collaborations to ensure fair and equitable drug access created with AI assistance.
It’s universally acknowledged that AI’s far-reaching influence extends well beyond mere convenience and efficiency; it stands as the bedrock of innovation in our contemporary digital realm. However, the potential of AI hinges on data, and the ethical handling of data is non-negotiable. This is where Unified Data Management (UDM) emerges as the linchpin of responsible AI development. UDM ensures that data is not only handled securely but also ethically, thereby safeguarding privacy, compliance, and user trust. It paves the way for what we call a transformative pitch in the AI ecosystem. In this context, Data Dynamics signifies a paradigm shift where the dynamism of data is harnessed to create a virtuous cycle of responsible AI, with UDM at its core, ensuring data’s integrity and ethical use.
The Data Dynamics Advantage
Unlock the full potential of AI while upholding ethical standards with Data Dynamics’ Unified Data Management (UDM) Platform. Our innovative solution is designed to empower companies on their journey to AI applications, serving as the ethical cornerstone of data-driven decision-making. Comprising four essential modules – Data Analytics, Mobility, Security, and Compliance – our platform integrates automation, AI, ML, and blockchain technologies. With a successful track record in over 28 Fortune 100 organizations, Data Dynamics scales seamlessly to meet global enterprise workloads. Say goodbye to fragmented data views and disparate solutions – with Data Dynamics, you can embrace a unified software platform that streamlines unstructured data management, proactively mitigates risks, safeguards sensitive data, and ensures comprehensive data governance. Embrace our propreitory
4S-Data Approach for Simplified, Secure, Strict, and Smart data management, leading to informed decision-making and a complete data transformation.
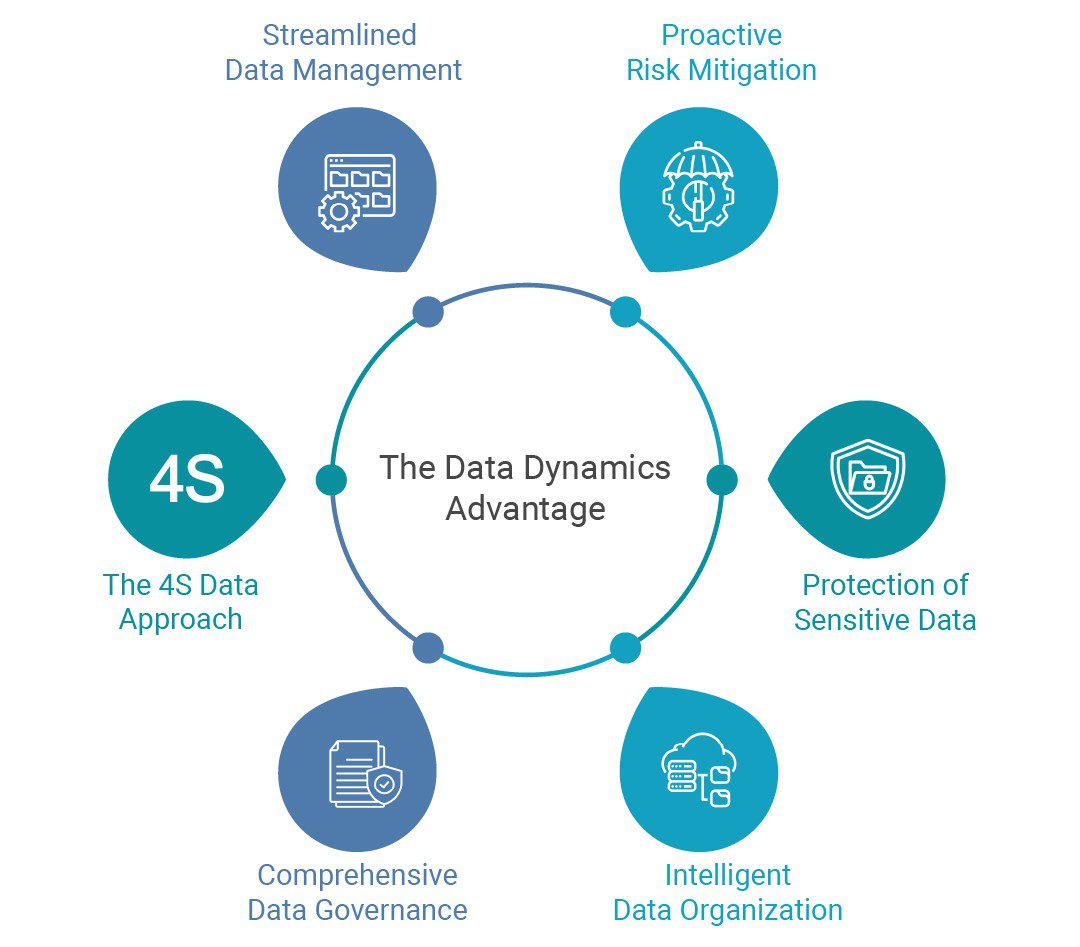
- Streamlined Data Management: Harness the power of a unified platform to seamlessly integrate compliance, security, and unstructured data management, creating a holistic AI journey.
- Proactive Risk Mitigation: Identify potential risks within unstructured content early on, empowering proactive risk management and compliance adherence.
- Protection of Sensitive Data: Utilize AI/ML-powered content analysis and a Data Science Engine to reliably detect and address PII and sensitive data.
- The 4S Data Approach: Embrace a Simplified, Secure, Strict, and Smart approach to extract invaluable insights from enterprise data in a secure, regulated, and optimized manner.
- Comprehensive Data Governance: Attain deep data visibility, stringent compliance adherence, and unchangeable audit reporting through blockchain for optimal data governance and informed decision-making.
- Intelligent Data Organization: Facilitate intelligent data organization with advanced file identification and categorization, secure storage, compliance classification, seamless data migration, and reporting, driving a complete data transformation in your AI journey.
With Data Dynamics, you’re not just managing data; you’re harnessing its true potential while ensuring ethical AI usage. To know more, visit www.datadynamicsinc.com or reach out to us at solutions@datdyn.com or (713)-491-4298.