Highlights:
- The evolution of cloud services from mere storage and processing solutions to adaptive cloud architectures is revolutionizing how enterprises approach AI workloads, dynamically tailoring resources and revolutionizing cost efficiency.
- Moving beyond a simplistic “lift and shift” approach, enterprises are urged to adopt a strategic and data-driven approach to unlock the full potential of the cloud, optimizing applications, and considering the intricacies of the data landscape.
- Caution is advised against overlooking critical aspects such as data optimization, potential biases in AI training data, and excessive use of file storage. A proactive data-driven hybrid cloud strategy is essential for successful and efficient cloud migration.
- The strategic partnership between Hitachi Vantara and Data Dynamics offers a comprehensive Data-Driven Cloud Adoption Solution, emphasizing advanced data visualization, elevated data utilization, robust data governance, and security measures to enable intelligent, secure, compliant, and optimized cloud adoption.
In this Blog:
In the ever-evolving realm of technology, the convergence of cloud technology is not merely a passing trend—it’s a transformative force reshaping the landscape of innovation for enterprises. The traditional perception of the cloud as a storage and processing solution has transcended, giving rise to a strategic frontier known as “Adaptive Cloud Architecture.” This paradigm shift goes beyond optimizing computational power; it’s a game-changer that unleashes the full potential of cutting-edge solutions without financial constraints.
Additionally, the “AI Ecosystem Cloud” and the creation of an “AI Innovation Hub” within the cloud are set to dismantle traditional barriers, fostering an environment where different models collaborate seamlessly, accelerating progress and fostering breakthroughs. However, amidst these exciting developments, a cautious approach is essential. The simplistic “lift and shift” methodology, without optimization, poses risks that may impede the realization of the full benefits of cloud adoption, particularly in the realm of data optimization.
This blog sets the stage for a comprehensive exploration of data-driven hybrid cloud adoption, offering insights into its significance and impact on enterprises. It navigates through the challenges of migrating data to the cloud, emphasizing the need for a strategic, data-driven approach. The blog also highlights the strategic partnership between Hitachi Vantara and Data Dynamics, aiming to revolutionize cloud migration and pave the way for a future where organizations thrive in the data-driven era.
Join us on this journey as we unravel the intricacies of a holistic Data-Driven Hybrid-Cloud Adoption that goes beyond migration—it’s about maximizing the cloud’s potential to drive innovation, efficiency, and success in the technology-driven era.
What is data-driven hybrid cloud adoption? How is it helping enterprises?
Moving data to the cloud, especially unstructured data, raises three important questions: What data are we moving, where is it going, and is it secure and compliant? These questions are crucial for success, covering key aspects of AI, from managing data and ethical considerations to cost-effectiveness. Let’s take a closer look.
At the heart of AI is the quality of its training data. Moving volumes of data without a deep understanding can significantly impact AI outcomes. So, businesses need to carefully curate and manage their data, ensuring accuracy and relevance for robust AI model training. This careful approach not only prevents potential biases but also establishes a strong foundation for the integrity of AI systems.
Enterprises also face the challenge of not having enough visibility into their data contents, often leading to unawareness of sensitive information within. The conventional lift-and-shift methodology in cloud migration may inadvertently overlook critical data, such as Personally Identifiable Information (PII) or Protected Health Information (PHI), potentially exposing it during AI training, resulting in severe repercussions. Success in a Hybrid Cloud strategy relies on a data-driven approach, requiring a comprehensive understanding of the data landscape, careful handling of sensitive information, and using insights for well-informed decisions.
Moreover, a common stumbling block in traditional cloud migration is the excessive use of file storage over object storage. While file storage is necessary in certain cases, its overuse can be more costly than the scalable and cost-effective alternative—object storage. Object storage, known for its scalability, is particularly fitting for managing unstructured data associated with AI applications. Blindly sending all data types to the cloud, especially relying on file storage, risks unnecessary consumption of storage space, perpetuating the misconception that the cloud is inherently expensive.
Seven Key Benefits of Data-Driven Hybrid Cloud Adoption
Effectively addressing these challenges requires adopting a practical, data-driven hybrid cloud strategy. This strategic approach involves the smart use of both on-premises and cloud-based infrastructure, guided by insights derived from a thorough analysis of the organization’s data needs and workload requirements, thereby creating an environment that is not only flexible and scalable but also optimized for efficiency. This proactive strategy not only tackles the complexities of AI success but also sets the stage for efficient data management in the cloud computing era, ensuring a smooth integration of data quality, security, and cost-effectiveness. As businesses navigate the digital landscape, this holistic approach guarantees a harmonious synergy of technological advancements and strategic foresight. Here are seven reasons why.
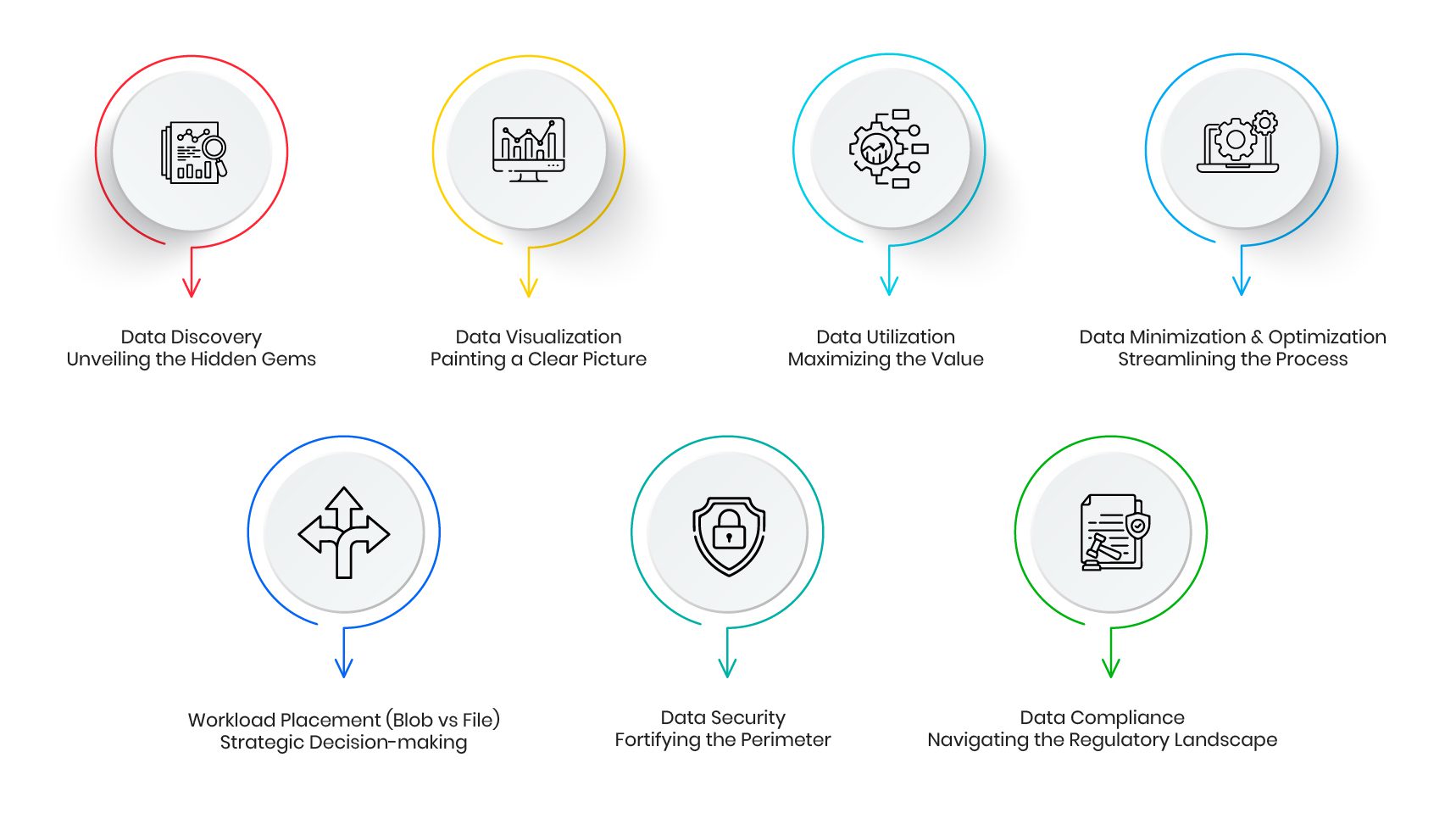
1. Data Discovery: Unveiling the Hidden Gems
Data discovery extends beyond mere identification; it involves an in-depth understanding of data structures, formats, interdependencies and sensitivity. The use of automated scanning tools, enhanced with machine learning algorithms, allows organizations to classify and catalog unstructured data effectively and safeguard consumer and business sensitive data. Techniques such as Natural Language Processing (NLP) and pattern recognition contribute to a more nuanced discovery process.
Addressing the challenges of unstructured data requires advanced algorithms for the detection and mitigation of Redundant, Obsolete, and Trivial (ROT) data. Employing hashing functions, metadata analytics, content analysis, and machine learning models enables the identification and elimination of duplicate and obsolete files, ensuring that only relevant and optimized data is slated for migration.
According to a study by Gartner, organizations that implement advanced data discovery techniques can reduce migration times by up to 30%, significantly enhancing overall project efficiency.
2. Data Visualization: Painting a Clear Picture
Effective data visualization transcends basic charts and graphs; it involves advanced techniques like context analysis and multidimensional scaling to depict intricate relationships within the data, facilitating dynamic visualizations and aiding stakeholders in identifying patterns, outliers, and anomalies.
Clustering algorithms play a pivotal role during the visualization phase by grouping related data, providing a more granular understanding of data interconnectivity. This not only streamlines the identification of dependencies but also contributes to the development of a migration roadmap based on the intrinsic relationships within the data.
3. Data Utilization: Maximizing the Value
Maximizing data utility requires a strategic combination of categorization, prioritization, and predictive modeling. Machine learning models integrated with data utilization strategies help predict data importance and usage patterns. This empowers organizations to make informed decisions regarding data retention, archival, and disposal, contributing to more efficient and purpose-driven migrations.
Implementing tiered storage solutions based on data temperature (hot, warm, cold) ensures that frequently accessed data is stored in high-performance environments, while less critical data can be moved to cost-effective storage options. AI-driven analytics tools, equipped with anomaly detection capabilities, play a crucial role in extracting meaningful insights from both structured and unstructured data, contributing to overall data optimization.
According to IDC, organizations that effectively prioritize data based on AI relevance can experience a 25% increase in AI model performance, leading to more accurate predictions and insights.
4. Data Minimization & Optimization: Streamlining the Process
Data minimization involves advanced optimization techniques to remove redundancies and unnecessary elements. Compression algorithms effectively reduce the size of data without compromising integrity. Deduplication techniques and employing algorithms can identify and eliminate duplicate data, further reducing the overall data footprint.
Efficient indexing, utilizing techniques like B-trees, aids in faster data retrieval. By optimizing data storage at a granular level, enterprises can achieve not only cost savings but also improved AI application performance through faster data access and processing.
5. Workload Placement (Blob vs File): Strategic Decision-making
Choosing between Blob and File storage involves a deep understanding of data characteristics and the specific requirements of AI workloads. Blob storage is ideal for unstructured data like images, videos, and documents, offering scalable and cost-effective solutions. File storage, utilizing protocols such as SMB or NFS, is suitable for structured data requiring shared access.
Data-driven insights derived from workload profiling help organizations make strategic decisions on the placement of different types of data. This ensures that AI workloads receive optimal support in terms of performance, scalability, and cost-effectiveness, taking into account the unique requirements of each workload.
6. Data Security: Fortifying the Perimeter
Data security in a hybrid cloud environment demands a multi-layered approach safeguarding consumer and business sensitive data. Implementing encryption algorithms like AES ensures data confidentiality during both storage and transmission. Access controls, using technologies like OAuth or LDAP, restrict unauthorized access, and continuous monitoring with Intrusion Detection Systems (IDS) helps identify and respond to security threats in real-time.
Advanced threat detection mechanisms, employing machine learning models trained on historical security data, enhance the ability to proactively identify potential security breaches. Integrating Security Information and Event Management (SIEM) tools provides centralized visibility into security events, aiding in incident response and compliance reporting. Additionally, the implementation of Zero Trust Security Models ensures that every access attempt is validated, even within the cloud environment.
According to a Ponemon Institute report, organizations that invest in robust data security measures experience a 40% reduction in the likelihood of a data breach during migration.
7. Data Compliance: Navigating the Regulatory Landscape
Adhering to data compliance regulations requires continuous monitoring and proactive measures. Automation tools, utilizing policy enforcement frameworks, ensure that data management practices align with evolving compliance requirements. Regular audits, facilitated by automated reporting mechanisms, provide evidence of compliance to regulatory bodies.
Blockchain technology, with its immutable ledger, contributes to data integrity, ensuring that compliance records are tamper-proof. Additionally, implementing Data Loss Prevention (DLP) solutions assists in preventing inadvertent data breaches, safeguarding sensitive information and maintaining regulatory compliance. By employing advanced consent management systems, organizations can ensure that they are collecting, storing, and processing consumer data in accordance with privacy regulations.
Data-Driven Cloud Adoption with Hitachi Vantara and Data Dynamics’ Solutions
Hitachi Vantara and Data Dynamics proudly announce a strategic partnership aimed at delivering a Data-Driven Cloud Adoption Solution—intelligent, secure, compliant, and optimized. By gaining insights into their data and building a robust cloud foundation, organizations can facilitate efficient and effective cloud adoption, reduce risk, ensure compliance, and enable unparalleled scale and compute power.
At the core of this transformative alliance lies Data Dynamics’ Analytics Software, tailored to address three pivotal aspects crucial for the success of cloud migration. Firstly, leveraging advanced data visualization, the software extracts actionable insights, providing decision-makers with a dynamic view of their data landscape. This not only enhances optimization but also facilitates informed decision-making, steering organizations towards more efficient operations and resource utilization throughout the migration journey.
Secondly, the software places a strong emphasis on elevating data utilization, availability, and accessibility. By simplifying data access and utilization, it not only breaks down silos but also ensures that information is readily available to support the migration process. This not only enhances productivity but also fosters collaboration across different teams, creating a more agile and responsive organizational environment during the critical transition to the cloud.
Moreover, the software goes beyond traditional measures by proactively addressing the challenges of data sprawl and cyber resilience. Robust data governance and security measures are seamlessly integrated to reduce risks associated with unauthorized access, data breaches, and cyber threats. This comprehensive approach not only safeguards sensitive and consumer data but also ensures regulatory compliance, providing organizations with a secure foundation as they embark on their cloud migration journey. In addition to these core aspects, the software introduces intelligent data placement to streamline resource allocation in the cloud environment. These advanced capabilities empower organizations to navigate the evolving landscape of digital transformation with confidence and agility.
This partnership seamlessly integrates with Hitachi Vantara’s existing suite of storage solutions, fostering a collaborative approach to meet the specific needs of customers. What sets this collaboration apart is its consultative and strategic approach, crafting tailored data management solutions for each company’s unique requirements. The process involves a meticulous analysis of stored data, generating insights for comprehensive risk assessment, ensuring compliance, and enhancing security. The ultimate aim is to transition data into a well-managed, secure, and efficient state.
As organizations embark on the dynamic journey of modern digital transformation, the Hitachi Vantara and Data Dynamics partnership stand as a beacon, guiding them toward a future where cloud migration is not just a transition but a strategic opportunity. Together, they are reshaping the cloud migration narrative, empowering organizations to thrive in the data-driven era and unlocking new possibilities for innovation and growth.
For further information or inquiries, please click here or contact us via email at solutions@datdyn.com. Additionally, you can schedule a meeting with one of our executives to explore the intricacies of the partnership and discover how it can enhance benefits for your enterprise. Set up a meeting by clicking here.